The next generation of CCaaS is here
Digital-first customer service, enterprise-scale voice support. Redefine customer service with an AI-powered platform that unifies voice, digital and social channels. Power channel-less interactions and seamless resolution no matter the channel of contact.
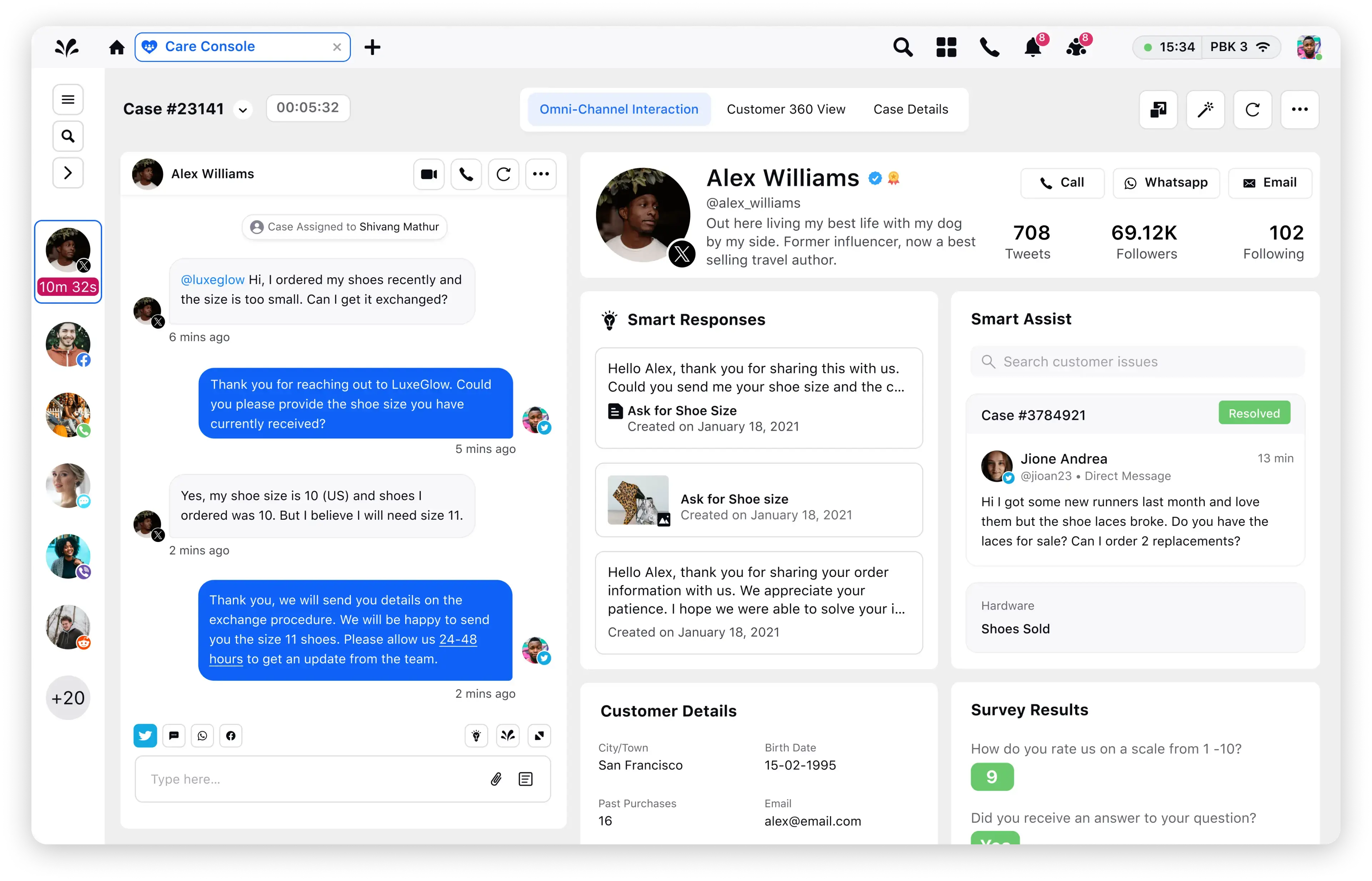
How to automate agent service quality management using AI
Agents are the first line of contact with the customers, and they can make or break a customer's experience. To safeguard the brand's reputation and to give customers a positive experience, you need an ongoing agent quality management process.
According to PwC, one in three consumers will walk away from a brand they love after just one bad experience
Therefore, quality audits are more than simply a necessity for every organization; they are a source to learn how well or poorly the agents are representing the brand.
Although quality control is crucial, doing it manually is tedious and time-consuming. It is not feasible for most organizations to sift through tens of thousands of case conversations to examine instances of hostile and friendly interactions.
- 4 major quality assurance pain points
- Benefits of leveraging AI in Quality Assurance
- Get visibility into 100% of case conversation
- Save time and resources
- Eliminate inconsistency and personal biases
- Base decisions on analytics and aggregate-level insights
- Enhance the customer experience
- How Sprinklr AI-powered agent scoring works
- Final thoughts
4 major quality assurance pain points
Benefits of leveraging AI in Quality Assurance
AI agent scoring automates the QA process by using the power of artificial intelligence and machine learning. It not only saves time and lowers costs, but also enables a thorough review of 100% of cases in addition to its comprehensive analytic capacity for giving contextual insights thus increasing efficiency without a horde of specialists. With AI-powered agent scoring you can:
Get visibility into 100% of case conversation
Traditionally, organizations sample case conversation with an average of 1-2% cases based upon metrics like low CSAT or low NPS and extrapolate its result to larger data. To make any decision based upon such a small sample can be misleading. In contrast, the AI model will automatically score each and every case as soon as it gets closed, giving visibility into 100% of case - conversation.
Save time and resources
During manual Quality management, supervisors have to read entire customer-agent interactions to gauge the quality of the agents. This means that Quality managers/supervisors will have to invest a lot of man-hours to gain agent insights. Using AI NLP models, these man-hours can be drastically reduced. AI will analyze the entire case conversation and will then assign a score across a number of parameters according to the guidelines.

Eliminate inconsistency and personal biases
Multiple analysts contributing to the scoring process raises the possibility of subjectivity, which can result in inconsistency. Also human intervention leaves room for personal biases. On the contrary, AI scoring removes human involvement and hence consistent as well as unbiased results are generated
Base decisions on analytics and aggregate-level insights
Using unified dashboards and artificial intelligence one can identify performance issues and actions needed to improve the customer experience. With these dashboards, it is easy to get a comprehensive perspective of an agent's strengths and limitations. This information can then be utilized to coach the agents by giving in target and personalized feedback, filling in critical talent gaps and hence enhancing the client experience.
Enhance the customer experience
Contact center teams can examine the elements that lead to negative customer experience using AI powered voice of customer and sentiment analysis. Indicators of unfavorable customer experiences, such as ineffective troubleshooting, escalations, Legal Issues etc. can be quickly identified and supervisors can then take actions to improve these flows to enhance customer experience.
How Sprinklr AI-powered agent scoring works
1. Inbound closed cases from various channels (Social, Digital, Email, Voice) are taken into the account.
2. AI scoring engines then runs on all the closed case conversations:
- It evaluates agents on both soft and hard skills
- Hard skills aid in evaluating operational efficiency, while soft skills help in evaluating the relationship that agents form with consumers.
- Parameters used to evaluate Soft skills are: empathy, courtesy, patience, proper opening and closing etc.
- Parameters used to evaluate hard skills are: promptness, efficiency, grammar, effective troubleshooting etc.
- Agent Quality score is calculated for each case by combining the scores across individual categories.
3. Unified dashboards providing aggregate level insights to make informed decisions
- Various customisable dashboards like areas of strength and improvements , channel wise AI score split, agent leaderboard are integrated for supervisors/quality managers through which performance gaps and concerns can be identified. These insights can be utilized to design an action plan for personalized coaching
- Multiple team leaders can also check the performance of their team with the help of these integrated dashboards
Final thoughts
Integration of AI in contact centers is driving a significant change in the way quality is managed and monitored. Supervisors and Quality Managers use to spend a lot of man-hours going through the customer-agent interactions to conduct audits. Nowadays, organizations are switching to Contact Center as a Service (CCaaS) for its AI-powered mechanism easy to scale and integrate with existing system.
All these interactions of contact center (Speech and Text) can now be analyzed with the AI capabilities which will provide insights related to agent performance and customer sentiment in very less time.
These insights can be then consumed by the supervisors to strategically plan the coaching sessions for their agents and track the improvements of the agents time over time.
This will not only improve the operational efficiency of the contact centers but will also enable the supervisors and quality managers to execute more strategic business decisions.
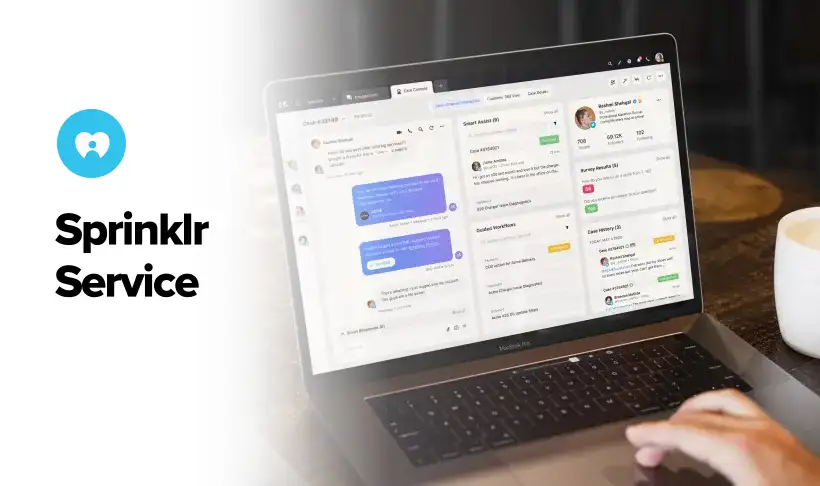