The next generation of CCaaS is here
Digital-first customer service, enterprise-scale voice support. Redefine customer service with an AI-powered platform that unifies voice, digital and social channels. Power channel-less interactions and seamless resolution no matter the channel of contact.
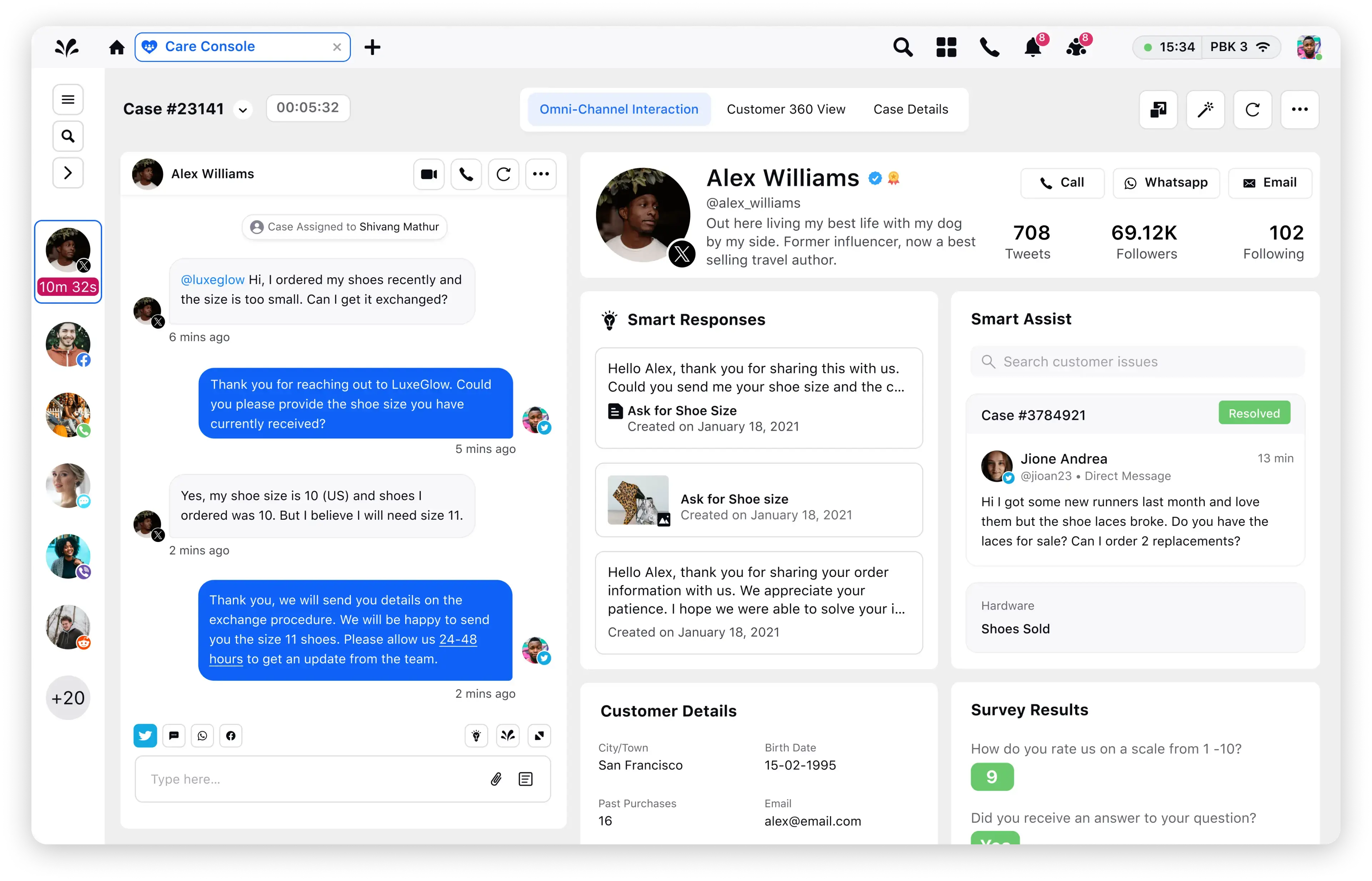
Machine Learning in Call Centers: A Guide to Get Started
The pressure on call centers to deliver faster, smarter, and more personalized service has never been greater. Customers expect instant solutions, minimal friction, and seamless interactions across channels — and they’re quick to move on if those expectations aren’t met. Call center machine learning is making its mark here, helping enterprises turn these challenges into opportunities.
With rising customer expectations and increasingly complex operations, businesses are leaning on machine learning to deliver what matters most — speed, accuracy and insights that drive exceptional customer experiences. After all, satisfied customers are not only loyal but also more profitable. They spend up to 140% more than their less satisfied counterparts.
For call centers, this means embracing ML to predict customer needs, streamline workflows and enhance real-time decision-making. In this blog, we’ll discuss real-life examples of machine learning in action, showcasing how it’s transforming call center operations. Also, learn the challenges and some quick tips on how to get started.
- What is the need for machine learning in call centers?
- Key applications of machine learning in call centers
- Real-life examples of machine learning in call centers
- Challenges in implementing machine learning in call centers
- How to get started with machine learning in your call center
- Power up your call center with best-in-class AI
What is the need for machine learning in call centers?
Enterprise-level call centers face growing challenges that make delivering exceptional customer service increasingly complex. High call volumes often result in extended wait times, while limited personalization can leave customers feeling undervalued.
Another critical hurdle is workforce management—balancing staffing levels with fluctuating demand is no small feat. Operational inefficiencies caused by manual processes and outdated systems further hinder performance, negatively impacting agent productivity and customer satisfaction.
This is where call center machine learning steps in, directly addressing these challenges by:
· 🤖 Automating routine tasks: Machine learning streamlines repetitive functions such as call routing, ticket categorization and response suggestions. This allows agents to focus on resolving complex customer issues, enhancing both productivity and outcomes.
· 🎯 Enhancing personalization: ML enables hyper-personalized interactions — tailored product recommendations, sentiment-based responses, and more by analyzing vast amounts of customer data. These interactions drive higher satisfaction and loyalty.
· 📈 Optimizing workforce management: ML-powered predictive analytics help managers create smarter schedules, matching staffing levels with demand peaks and reducing the risks of overstaffing or agent burnout.
· ⚙️ Improving operational efficiency: Machine learning eliminates bottlenecks by automating processes, detecting anomalies, reducing manual errors, and ensuring smoother call center operations.
The impact is undeniable. According to Statista, 57% of businesses already use machine learning in customer service to enhance customer experiences. Call centers embracing ML report improved customer satisfaction (CSAT) scores, increased agent productivity, and shifted from reactive problem-solving to proactive customer engagement.
Key applications of machine learning in call centers
Machine learning is revolutionizing call center operations, especially for enterprise-scale organizations managing vast volumes of customer interactions daily. By integrating intelligent technologies, businesses can deliver faster, more personalized and highly efficient customer service. Below, we explore one of the most impactful applications of call center machine learning:
1. Automated call routing
ML algorithms analyze customer interactions (voice, text, chat, etc.) to understand their intent, sentiment, and urgency. They enable intelligent routing of calls to the most suitable agents (e.g., based on skills, language, and availability). Directing calls to the right agent from the start significantly reduces call resolution times. Faster resolutions and reduced transfers lead to happier customers.
2. Sentiment analysis
Through call center machine learning, you can analyze tone, language, and context to identify customer sentiment during interactions. Identifying frustrated or angry customers allows agents to address concerns and prevent escalation proactively. Sentiment analysis data can also be used to identify areas for improvement in call center agent training and coaching.
3. Predictive analytics
ML algorithms analyze historical data (call volumes, contact center agent performance, and customer behavior) to forecast future trends. Predicting call volumes accurately helps optimize staffing levels, minimize wait times, and maximize agent utilization. Plus, it helps identify potential service disruptions and proactively allocate resources to prevent issues.
Jennie Maze Limited, a rapidly growing insurance company, faced challenges in managing its call center operations, including high call volumes, uneven agent workload distribution and difficulty predicting customer needs. The company implemented a comprehensive predictive analytics strategy focusing on time series forecasting to address these issues. This data-driven approach enabled Jennie Maze Limited to proactively anticipate customer needs, optimize agent scheduling and improve overall customer satisfaction.
4. Conversational AI and chatbots
AI-powered chatbots can handle a wide range of customer inquiries (e.g., answering FAQs, providing product information, and processing orders) 24/7. By effectively handling routine inquiries, chatbots significantly reduce the volume of calls to the call center. They also empower customers to find answers and resolve issues by suggesting relevant knowledge bases, enhancing their self-service experience.
Vodafone introduced an AI chatbot named TOBi to manage routine customer queries. This resulted in a 68% reduction in call volumes and a 43% increase in customer satisfaction ratings.
5. Real-time agent assistance
ML-driven tools provide agents with real-time guidance during calls, such as suggested responses or next-best actions based on customer input. This boosts efficiency and ensures consistent service quality.
HotelPlanner, a leading hotel booking platform, introduced AI-powered travel agents to handle customer inquiries. These AI agents managed over 40,000 holiday inquiries and generated £150,000 room reservations within their first month. By operating 24/7, the AI agents doubled call volumes and significantly increased bookings, demonstrating the effectiveness of AI in enhancing customer service and operational efficiency.
😊 Good to know
Sprinklr’s AI-powered Agent Assist software takes real-time guidance to the next level. By providing tailored recommendations, including personalized responses, relevant knowledge base articles and optimal next actions, Sprinklr helps agents focus entirely on the conversation. This minimizes average handling time and maximizes customer satisfaction by delivering actionable insights precisely when needed.
Sprinklr’s AI-powered Agent Assist ensures agents can handle even the most complex conversations with confidence and agility, transforming every interaction into an opportunity to delight.
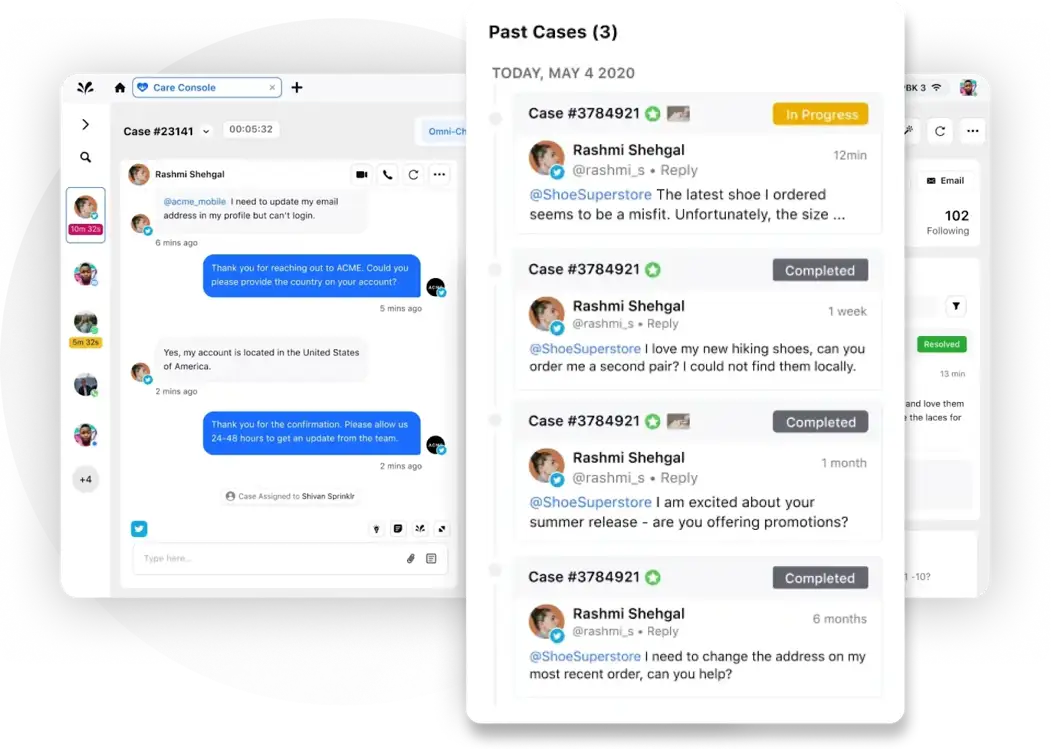
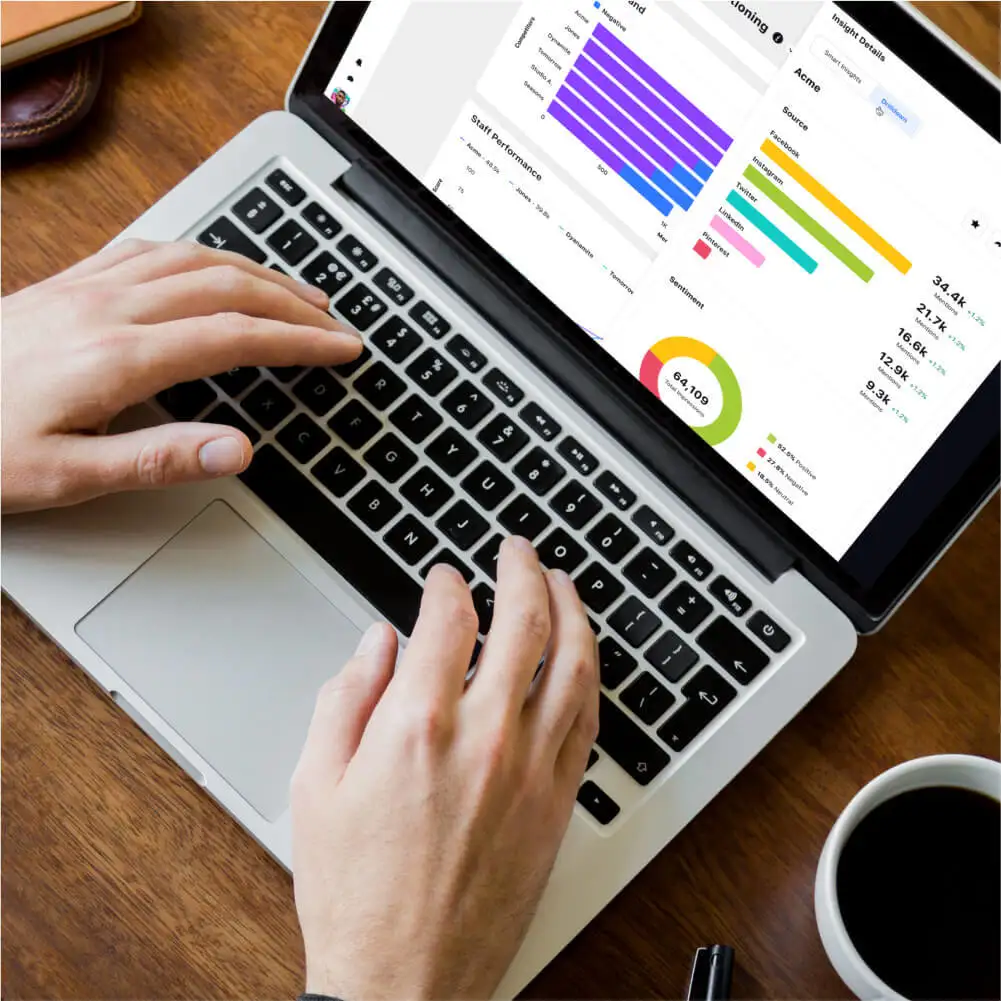
Real-life examples of machine learning in call centers
Here are a few real-life examples of how large enterprises leverage machine learning to transform their call center operations.
1. Bank of America
Bank of America harnesses predictive analytics to forecast call volumes and anticipate potential service disruptions. This proactive approach enables the company to optimize workforce management, ensuring smoother operations during peak times.
Additionally, the bank employs AI-powered call routing systems to analyze customer inquiries in real-time and direct calls to agents whose skills and expertise best match the customer’s needs.
The results have been remarkable — reduced average wait times, improved first-call resolution rates and enhanced customer satisfaction scores.
2. Delta Airlines
Delta Airlines utilizes sentiment analysis to monitor customer conversations across social media and other channels. Agents are provided with real-time alerts and insights into customer sentiment during interactions. They also receive real-time assistance, such as suggested scripts and knowledge base articles, to help them resolve customer issues effectively. This helped Delta Airlines improve customer satisfaction, reduce negative sentiment on social media and increase agent confidence and job satisfaction.
How Sprinklr helps
Imagine being able to identify why your customers are reaching out and proactively address their needs before they even voice them. With Sprinklr conversational analytics software, you can analyze customer intents and sentiments across 100% of your interaction data, uncovering emerging trends and opportunities.
Sprinklr goes beyond reaction — it empowers you to anticipate. With real-time analytics, you can spot potential issues before they escalate, safeguarding your CSAT scores. Intelligent auto-alerts from Sprinklr AI instantly notify supervisors of emerging concerns, ensuring no customer need slips through the cracks.
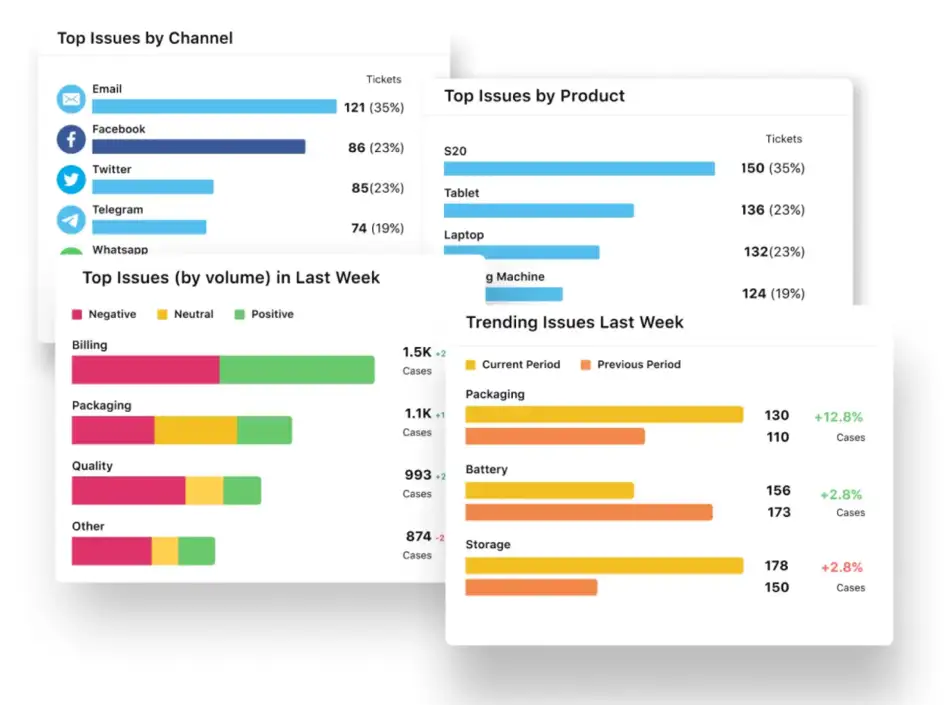
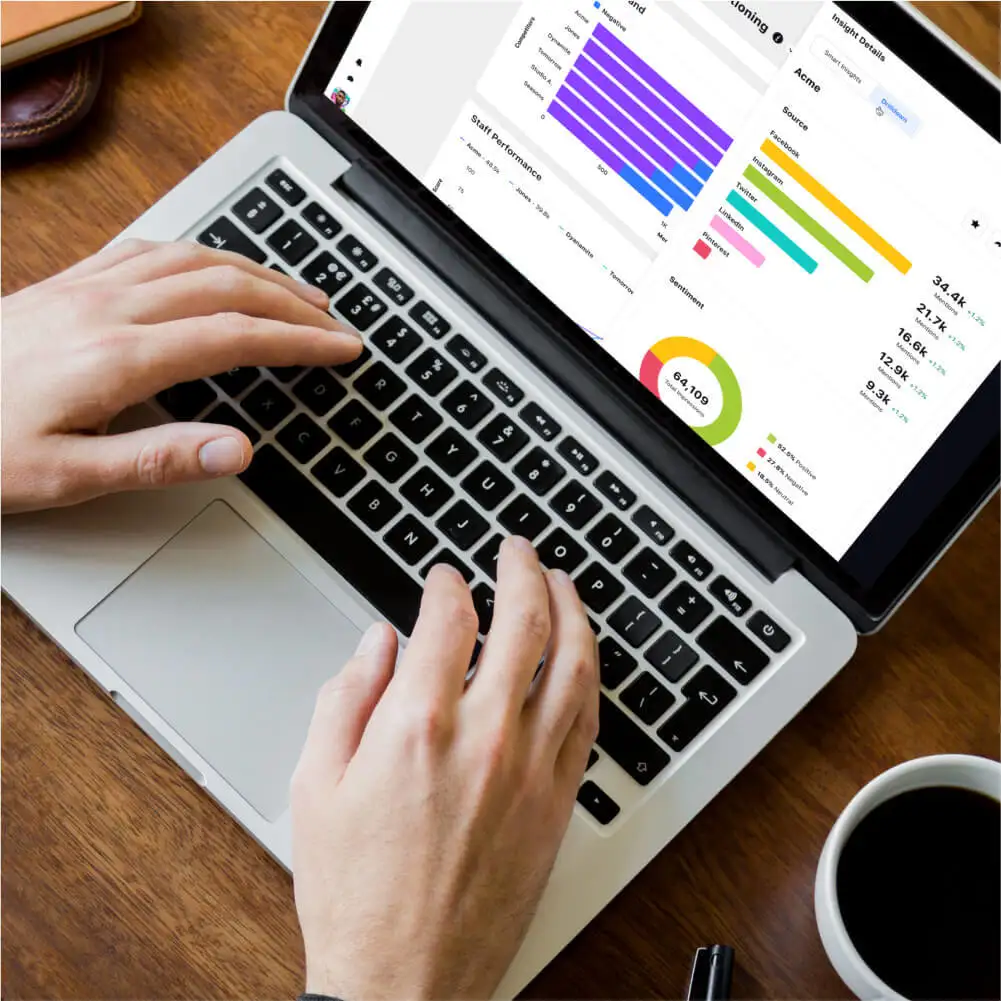
Challenges in implementing machine learning in call centers
While machine learning can potentially transform call centers, large enterprises often face significant challenges when implementing these advanced technologies. Here are some of the top hurdles they face.
🔐 Data privacy concerns
For large organizations, particularly those handling sensitive customer data, compliance with stringent data privacy regulations like GDPR and CCPA is paramount. Failure to uphold data security standards can lead to legal repercussions, financial penalties, and irreparable damage to a company’s reputation.
✅ Solution
The key to overcoming this challenge is prioritizing data encryption anonymization and ensuring compliance with local and international regulations. When choosing ML solutions, opting for those built with robust security frameworks in mind is crucial. Additionally, regularly scheduled audits are essential to maintaining data integrity and trust.
🖥️ Integration with legacy systems
Many enterprises rely on legacy systems not designed to work seamlessly with modern ML technologies. Integrating new AI-driven tools with these outdated systems can be time-consuming and expensive.
✅ Solution
A successful integration strategy involves a gradual, phased approach. Start by introducing ML solutions that can work alongside your current systems without requiring a complete overhaul. This allows for smoother transitions and reduces operational disruptions.
Over time, expand and integrate more advanced capabilities as your infrastructure evolves. Opt for scalable ML solutions that support incremental modernization, enabling you to upgrade your systems step by step without a major financial burden.
💰 High initial investment
Implementing machine learning at scale requires substantial upfront investment in technology, infrastructure and training. The cost can be prohibitive for large organizations, especially when results may take time to materialize.
✅ Solution
To mitigate this challenge, look for flexible pricing models that allow you to start small and scale as needed. This gives you the freedom to pilot ML solutions with minimal financial risk, testing their effectiveness before committing to full-scale deployment.
Additionally, partner with vendors who offer robust training and support. This ensures that your team can quickly get up to speed with the technology, making it easier to realize value from day one and accelerate time to ROI.
📚 Change management and employee training
Adopting call center machine learning requires a shift in mindset, especially for call center agents who are used to traditional workflows. Employees may resist new technology, fearing job displacement or the learning curve involved.
✅ Solution
To overcome this resistance, robust change management strategies that communicate the role of ML as a tool to augment rather than replace human agents are essential.
Frame the conversation around how AI enhances agents’ capabilities, helping them work smarter and more efficiently rather than making their jobs obsolete. Offer comprehensive training programs that teach employees how to use AI tools and emphasize the value these technologies bring to their daily work.
Editor’s Pick: Change Management in Call Centers: Key Checklists
How to get started with machine learning in your call center
Implementing machine learning in your call center doesn’t have to be overwhelming. By taking a strategic, step-by-step approach, you can harness the power of ML to optimize your operations and deliver exceptional customer experiences. Here’s how to get started.
1. Assess your current operations
Before diving into machine learning, it's crucial first to understand your call center's current state. Implementing machine learning in call centers can be a shot in the dark without a clear grasp of your existing technology, processes and workflows. Start with a comprehensive audit of your operations. Map out your key systems, identify existing pain points and review the currently available data.
Focus on key call center KPIs such as average handle time (AHT), first-call resolution (FCR), customer satisfaction (CSAT) and agent performance metrics. These will serve as benchmarks for measuring the impact of ML down the road. Look for bottlenecks or areas of inefficiency — whether it's long wait times, ineffective call routing or even high agent turnover.
But don't stop there—also consider strategic business goals. Where do you most need improvements? Do you want to increase agent productivity, enhance customer experience, or optimize resource allocation? By identifying specific pain points and aligning ML goals with business objectives, you ensure the technology delivers tangible benefits. This initial assessment sets the stage for a targeted, focused approach to integrating machine learning into your call center's operations.
2. Choose the right tools
Selecting the right machine learning tools is critical to ensuring your success. The right tool can unlock your call center’s potential, while the wrong one can lead to inefficiencies and customer frustration. Start by defining your objectives — improving call routing, automating tasks, or enhancing customer interactions. Once your goals are clear, look for solutions that align with those needs.
Evaluate tools based on their ability to scale with your operations. As your call center grows, your ML solution should seamlessly expand without requiring a complete overhaul. Prioritize tools that integrate smoothly with your existing platforms — this will help streamline implementation and reduce disruption. Whether adding predictive analytics, sentiment analysis or real-time agent assistance, the selected tools should complement and enhance your current systems, not replace them.
Additionally, consider future-proofing. Choose solutions flexible enough to adapt to advancements in ML and AI technologies, ensuring your investments remain relevant as innovation progresses.
Achieve 90%+ accuracy with Sprinklr AI
Sprinklr AI stands out as a comprehensive solution, offering a suite of capabilities designed to empower your team while optimizing customer experiences. With 90%+ accuracy, Sprinklr’s specialized AI models drive productivity and better decision-making, ensuring seamless service at every touchpoint.
- Empower your agents: Sprinklr AI boosts agent productivity and first-call resolution rates by optimizing responses for tone, relevance and accuracy. Agents can quickly extract answers from the knowledge base and automated case summarization and disposition ensure faster resolutions.
- Enhance self-service: With Conversational AI+, quickly deploy and scale generative AI-powered conversational bots. These bots provide deeply contextual and relevant responses, improving self-serve rates and streamlining customer interactions.
- Monitor and improve quality: Automate quality monitoring and receive recommended actions based on customized AI scoring parameters. This ensures your agents maintain consistent interaction quality while enabling smarter, data-driven decisions for continuous improvement.
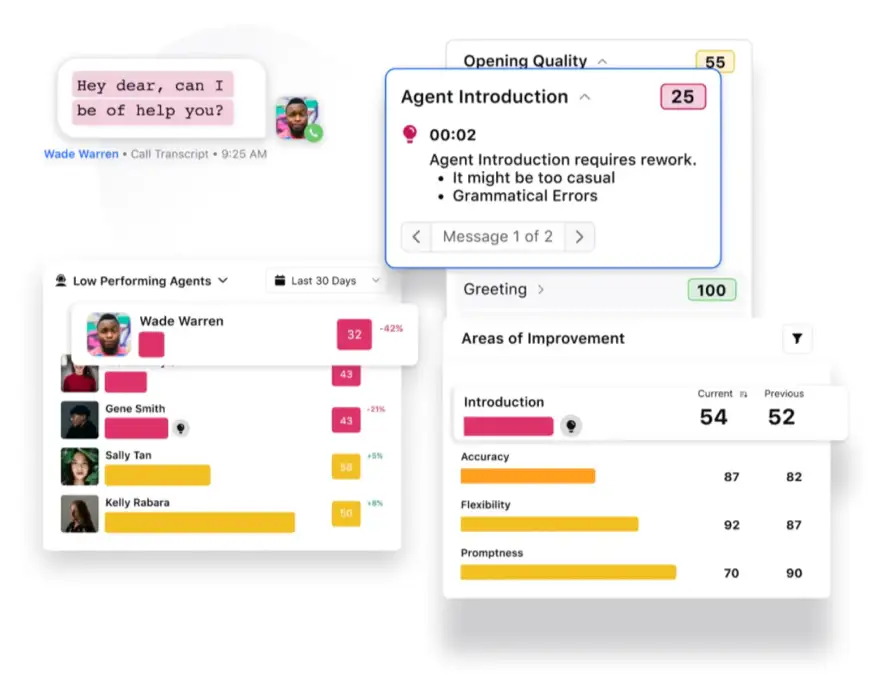
3. Build a data-driven culture
Machine learning thrives on data. Your business must embrace a data-driven culture to make the most of ML. Encourage teams to collect and analyze customer data to uncover insights that drive thoughtful decision-making.
🎯 Pro Tip
Promote ongoing data literacy training across your teams to ensure they can interpret and act on the insights provided by your ML models. Equip your agents with the tools and knowledge to leverage these insights during customer interactions — helping them tailor conversations, improve problem-solving and boost satisfaction. The more empowered your team is with actionable data, the more seamless and impactful the customer experience will be.
4. Start small with pilot projects
Rather than attempting a full-scale overhaul of your entire call center operations, it is wise to begin with pilot projects. Focusing on specific areas where ML can deliver quick wins, such as automated call routing, sentiment analysis, or predictive analytics, allows you to demonstrate the technology’s value in a controlled, manageable way. You can gradually build confidence in the technology by closely monitoring the results and iterating based on performance metrics and team feedback.
5. Monitor and scale
Once you've successfully run pilot projects and seen initial positive outcomes, it's crucial to focus on continuous monitoring of the ML models. This allows you to track real-time performance, identify challenges and make necessary adjustments. As your models evolve and your understanding deepens, regular optimization ensures that your ML tools align with your business goals.
Power up your call center with best-in-class AI
Let’s face it — call centers are no longer just about answering calls. They’re the frontline of customer experience, and if your business isn’t leveraging AI to improve operational efficiency, customer satisfaction and agent performance, you’re falling behind. The industry is evolving at lightning speed, and those who aren’t adapting to AI-driven solutions will inevitably struggle to keep up.
The companies that truly capitalize on AI are seeing dramatic improvements in customer satisfaction, operational costs, and agent productivity. But the road to AI adoption is challenging. Data privacy concerns, integration complexities, and high upfront costs can feel like major roadblocks. So, how do you overcome them without getting bogged down?
The answer is simple: partner with Sprinklr Service.
Sprinklr’s AI-powered solutions are built to solve the exact problems you’re grappling with. With Sprinklr Service, you’ll be able to:
- Empower your agents with real-time assistance to handle issues swiftly, reducing resolution times.
- Improve self-service with AI-driven chatbots and Conversational AI+.
- Continuously monitor performance with actionable insights to optimize your call center operations.
- Schedule a demo of Sprinklr Service today and see firsthand how it can revolutionize your call center operations.
Frequently Asked Questions
Machine learning can streamline call center operations by automating repetitive tasks, improving call routing and providing real-time insights. This can reduce wait times, enhance agent productivity and improve customer satisfaction in large-scale environments.
Machine learning can automate tasks such as call routing, sentiment analysis, data entry, customer support through chatbots and predictive analytics for forecasting call volumes, improving both efficiency and accuracy.
AI refers to the broader concept of machines performing tasks that would typically require human intelligence. In contrast, machine learning is a subset of AI that enables systems to learn from data and improve over time without explicit programming.
Automated call routing uses machine learning algorithms to analyze customer inquiries and route calls to the most suitable agent based on factors like customer history, sentiment and agent expertise, ensuring quicker and more accurate resolutions
Machine learning in call centers should prioritize customer privacy, ensure data security and be transparent in its decision-making. Ethical practices involve obtaining customer consent for data use, avoiding bias in AI models, and maintaining compliance with data protection regulations.