The next generation of CCaaS is here
Digital-first customer service, enterprise-scale voice support. Redefine customer service with an AI-powered platform that unifies voice, digital and social channels. Power channel-less interactions and seamless resolution no matter the channel of contact.
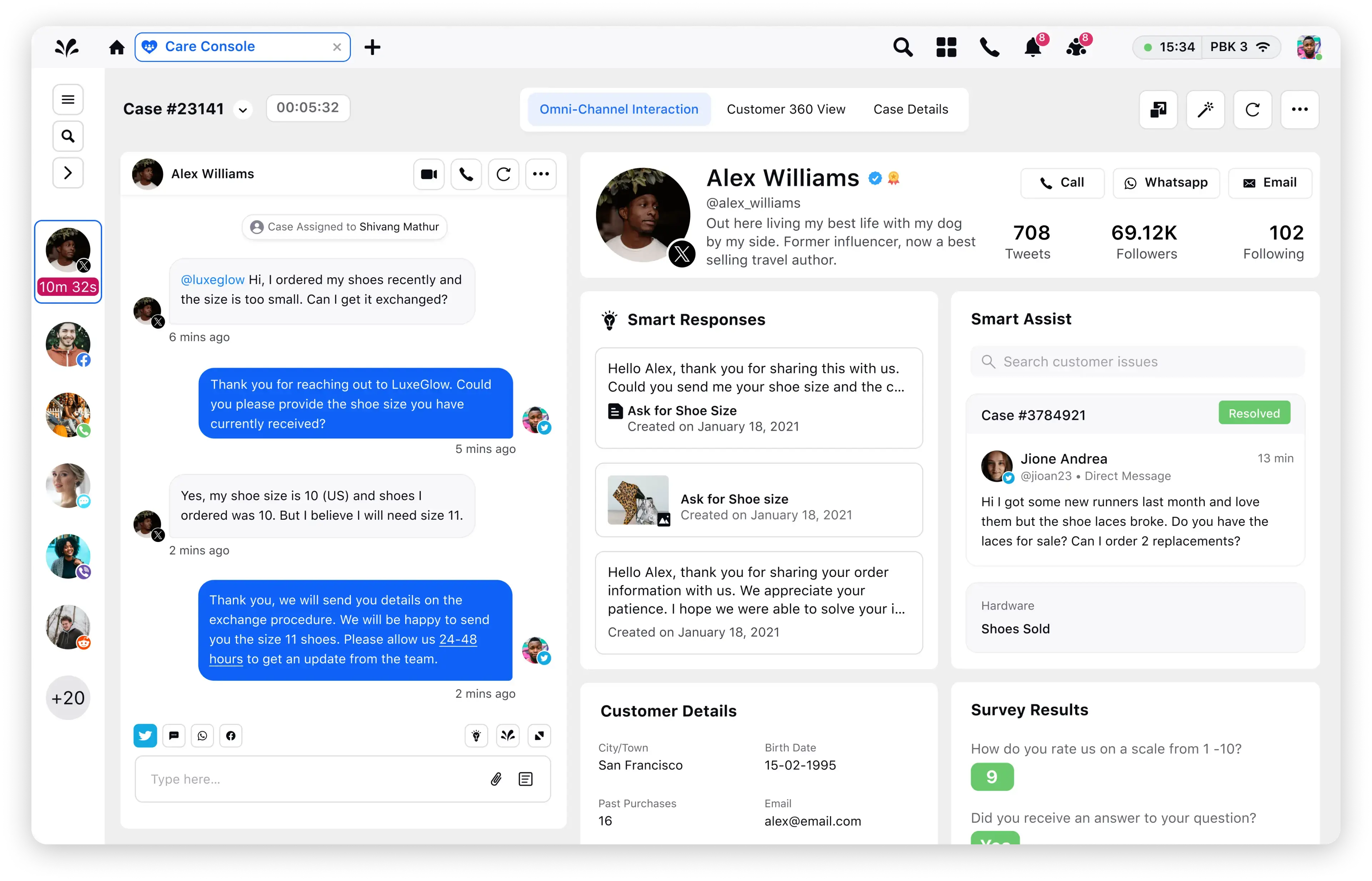
Customer Sentiment Analysis: How to Get Insights (+ Tools)
The days of clear product differentiation are long gone. With nearly every product replaceable and every service offering an alternative, the competition in today’s market is fierce. So, how do you stand out?
Well, by understanding what truly matters to your customers — their pain points, preferences and the nuanced moments that shape their experiences.
This is where customer sentiment analysis becomes invaluable. It identifies patterns in customer opinions, preferences and frustrations, offering a deeper understanding of their expectations. Research supports this: satisfied customers aren’t just loyal; they’re more profitable, spending up to 140% more than those who aren’t.
Even industry leaders see its growing importance. Lauren Villeneuve, Senior Director of Advisory at Gartner, highlights that methods for capturing the Voice of Customer (VoC) are evolving. By the end of 2023, 72% of customer service leaders are expected to pilot or adopt sentiment analysis solutions, emphasizing how businesses increasingly prioritize tracking customer feedback across multiple channels.
In this blog, we’ll delve into what customer sentiment analysis truly means, its different types and how it compares to emotion analysis. We’ll also explore the tools and strategies you can use to uncover actionable insights and create impactful customer experiences.
What is customer sentiment analysis?
Customer sentiment analysis is leveraging data, artificial intelligence (AI) and natural language processing (NLP) to assess customer emotions, opinions and attitudes toward your brand, product or service. Replacing guesswork with data-driven insights offers you a structured approach to measuring and responding to customer emotions.
Here’s how the process works:
1️⃣ Data collection: Sentiment analysis gathers data from various sources — social media, emails, chat transcripts, customer surveys and reviews. This broad spectrum ensures a well-rounded view of customer sentiment.
2️⃣ NLP and text analysis: Using NLP, the collected data is analyzed to identify emotional tones. It evaluates word choice, tone and phrasing to determine whether customer statements are positive, negative or neutral.
3️⃣ Sentiment scoring: Each piece of feedback receives a sentiment score on a defined scale, often ranging from -1 (negative) to +1 (positive). These scores give you an at-a-glance understanding of customer mood and help prioritize areas requiring attention.
4️⃣ Categorization by theme: Feedback is categorized into themes like “product quality,” “support response” or “pricing.” This thematic analysis allows you to pinpoint where you excel and where improvements are needed.
5️⃣ Real-time feedback: Advanced sentiment analysis tools offer live tracking capabilities, enabling immediate action when a customer’s sentiment takes a dip — an invaluable asset for customer support teams.
For example, consider a global e-commerce brand tracking customer support calls using sentiment analysis. The system can instantly notify supervisors if sentiment scores drop due to recurring complaints about delivery delays. Acting on this feedback — through enhanced delivery logistics or targeted support training — helps the brand address pain points proactively, improving customer satisfaction and reducing potential churn.
🔖 Check this out: 4 sentiment analysis examples to improve your customer experience
Types of client sentiment analysis
Customer sentiment goes beyond just knowing if feedback is positive or negative. The four main types of sentiment analysis — polarity-based, emotion-based, urgency-based and intention-based — help you dive deeper into the “why” behind customer feelings. Here’s a breakdown:
Type | Description | Elements detected | Example |
Polarity-based | Focuses on classifying sentiments as positive, negative or neutral. | Keywords, tone, context | A telecom company analyzing call transcripts considers statements like “This network is fantastic!” as positive, while “I keep losing signal” scores as negative. This quick polarity check helps it prioritize service improvement areas. |
Emotion-based | Detects specific emotions such as happiness, anger or frustration. | Emotional keywords, voice tone, context | In retail, a sentiment analysis tool might detect customer frustration in reviews with phrases like “I waited too long” or “disappointed.” Retail brands can refine their service by identifying and addressing this, reducing negative emotional triggers across customer touchpoints. |
Urgency-based | Identifies feedback or conversations that indicate a need for immediate action. | Urgent words (e.g., “now,” “ASAP”), context, intensity | A bank can use urgency-based sentiment to flag issues with phrases like “My account is locked and I can’t access funds!” as a high priority. This enables support teams to respond faster to prevent escalation, especially for critical issues like blocked transactions. |
Intention-based | Analyzes customer intent, such as a desire to buy, leave or seek support. | Intent phrases, action words | A software company could use intention-based sentiment to identify potential buyers or cancellations. For example, “I’m ready to upgrade” signals interest in purchasing, while “I might cancel if this issue continues” warns of potential customer churn. By targeting these signals, the company can proactively reach out with solutions to close a sale or retain customers. |
Customer sentiment analysis vs. emotion analysis
Customer sentiment and emotion analysis might sound similar, but each serves a distinct purpose and offers unique insights. Here are the key differences between them:
Aspect | Customer sentiment analysis | Emotion analysis |
Objective | To gauge the general sentiment — positive, negative or neutral — toward a product, service or brand. | Helps identify specific emotions (e.g., happiness, anger, fear) within customer interactions. |
Approach | Focuses on overall tone and context to classify sentiment, often assigning scores for positivity or negativity. | Detects emotional cues from language, tone and behavior to determine distinct emotions present in interactions. |
Core elements | Includes polarity (positive, negative, neutral), keywords, context and tone. | Identifies emotional keywords, vocal cues and intensity of language (words like “frustrated,” “excited,” “angry”, etc.). |
Applications | Understands brand perception, identifies trends and tracks satisfaction levels over time. | Helps assess the emotional state of customers during interactions, essential for personalized support and conflict resolution. |
Advantage | Provides a broad understanding of customer loyalty and satisfaction. | Enables deeper emotional insights, which can lead to empathetic customer engagement and improved customer relations. |
Why is consumer sentiment analysis important?
Consumer sentiment analysis offers a window into customer preferences and perceptions, making it an essential tool for modern businesses. Here are some of the major benefits it brings:
😊 Enhances customer experience
Understanding customer emotions is critical in an era where 89% of businesses compete primarily on customer experience. Sentiment analysis doesn’t just highlight areas needing improvement and uncovers what’s working well, offering a roadmap for delivering delight at every touchpoint.
For instance, if customers praise your team’s responsiveness but express frustration over insufficient product knowledge, sentiment analysis can flag the need for specialized agent training. By addressing such patterns, you can deliver highly personalized experiences that build loyalty and set your business apart in a crowded marketplace.
📊 Delivers actionable insights
Sentiment analysis goes beyond surface-level metrics, examining the underlying emotions and intent behind customer interactions. Phrases like “hard to use” or “would love” provide clear cues for improvement, helping you refine products, services or strategies in line with customer needs.
These insights can fuel product development, optimize customer support processes and drive strategic decisions. Companies that act on this feedback create offerings that resonate deeply with their audience, leading to higher satisfaction and stronger engagement.
🤝 Strengthens brand loyalty
Personalized customer experiences are a proven loyalty booster, with research showing that brands offering personalized experiences earn 1.5 times more loyalty than those that don't. Sentiment analysis enables this personalization by tuning into positive and negative customer feedback and demonstrating their voices are heard.
Addressing customer concerns — whether by adding a requested feature or speeding up service times — strengthens trust and reinforces the emotional bond between your brand and customers, driving long-term loyalty.
🎧 Watch Now: The Human Touch: How Personalised Experiences can Drive Business Growth
🌐 Identifies market trends
With sentiment analysis, you can tap into emerging customer preferences, revealing trends before they become mainstream. By evaluating social media posts, reviews and online discussions, you can spot shifts in demand and customize your offerings.
For customer service, this means anticipating and responding to changing expectations — perhaps investing in faster response times, new support channels or even AI-powered solutions. Being proactive keeps you relevant, positioning you as leaders who understand and adapt to customers' needs.
5 Ways to get insights through customer sentiment analysis
As a business, you must dive deep into customer emotions, opinions and intentions to grasp true customer sentiment. Let’s see how to uncover these insights.
1. Opinion mining
Opinion mining, or aspect-based sentiment analysis, breaks down customer feedback into specific components, such as features, service aspects or product attributes. It allows you to identify what customers love and what they want to improve.
AI-powered tools scan reviews, surveys, images, videos and social posts for specific mentions of key attributes (e.g., “battery life,” “customer support,” “ease of use”) and pair them with sentiment indicators.
For example, a global ride-hailing company may use opinion mining on customer app feedback. They may find overall sentiment positive, but mining reveals a recurring theme: customer dissatisfaction with driver communication. The company can address this by introducing real-time translation features in the app, creating seamless communication between drivers and riders in different languages.
Opinion mining allows you to focus on high-impact improvements with measurable outcomes, aligning investments with customer priorities.
2. Rule-based analysis
Rule-based sentiment analysis categorizes customer feedback using predefined rules — specific keywords, emojis or expressions — to deliver quick and structured consumer insights. It’s particularly useful if you seek low-complexity methods for immediate action.
For example, if you run an e-commerce company and your customers mention “delivery delay” or “couldn’t reach support,” the rule-based system categorizes these as negative sentiments and flags them for follow-up.
Similarly, a retail chain may use a rule-based system to monitor social media posts for terms like “refund issue” or “great customer service.” Negative posts trigger immediate outreach, while positive comments are routed to marketing for social proof campaigns.
The rule-based analysis method is particularly effective for identifying recurring issues, helping you to address common customer pain points in real-time.
📌 C-Suite Takeaway
Rule-based analysis is ideal for quick wins, especially in scenarios where speed is critical. For instance, during a product launch, tracking keywords like “issue,” “love” or “confused” across social media can help your teams respond in real-time, safeguarding customer trust.
However, to unlock deeper and more actionable insights, pairing this approach with reliable sentiment analysis tools discussed later in this blog can make all the difference.
3. Customer emotion analysis
Emotion analysis interprets the underlying emotional states in customer feedback, such as anger, happiness, frustration or excitement, offering a richer layer of understanding than simple positive, neutral or negative categorizations. By recognizing these emotions, you can pinpoint customer pain points or moments of delight and adjust your approach accordingly.
For instance, a luxury hospitality chain may use emotion analysis on post-stay surveys and call center interactions. It may identify heightened frustration among repeat guests about inconsistent check-in processes, leading the leadership to implement a “preferred guest” expedited check-in system, resolving the issue and reinforcing loyalty among high-value customers.
😊 Good to know
Sprinklr’s conversational analytics software enables real-time action by helping you understand the reasons behind your customers’ concerns. Rather than just identifying trending topics or customer intents, it analyzes sentiments across 100% of conversation data, providing valuable insights into what truly drives customer satisfaction or dissatisfaction.
The tool delves into specific contact drivers, revealing their underlying attributes, root causes, and actionable recommendations. This comprehensive approach ensures you proactively address issues, enhancing the overall customer experience.
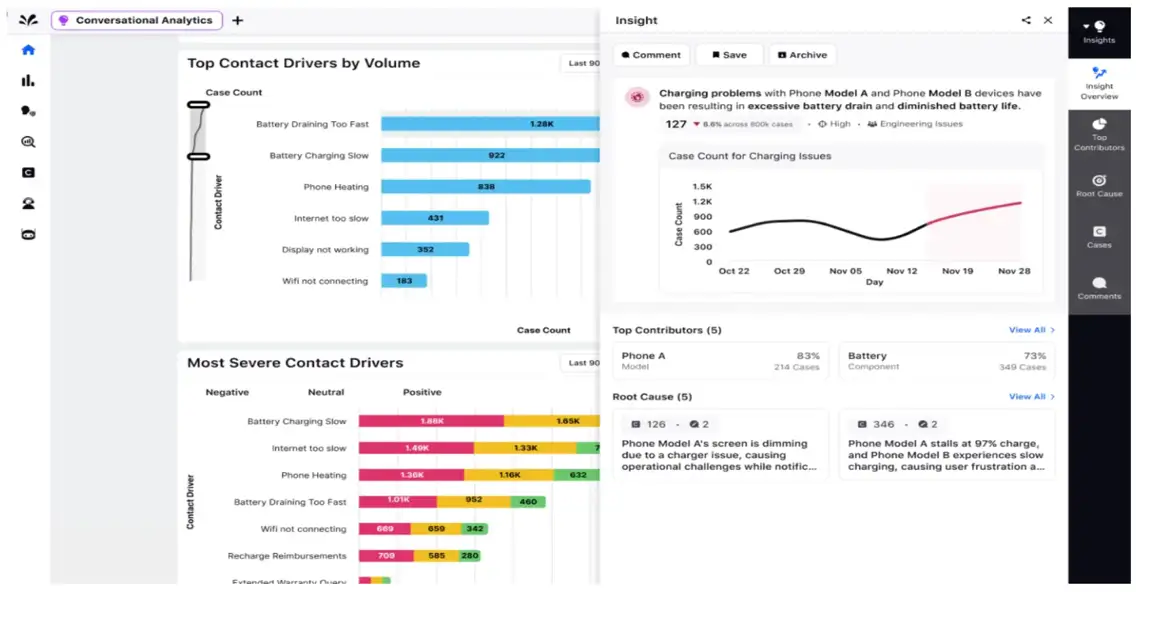
4. Multilingual sentiment analysis
In a global marketplace, customers interact with brands in various languages. Understanding their feedback without language barriers is essential. Multilingual sentiment analysis allows you to capture and interpret customer sentiments in multiple languages.
This approach helps brands gain authentic insights from customers worldwide, regardless of their language. For instance, a multinational consumer electronics brand may launch a new product and use multilingual sentiment analysis to assess its reception across regions. Insights reveal positive sentiment in North America about performance but customer dissatisfaction in Asian markets due to unclear user manuals. Leaders may implement region-specific updates, driving satisfaction globally.
💡 Quick Tip
Prioritize the most common languages your customer base speaks and ensure translation accuracy by using AI-driven language models that keep sentiment context intact.
However, avoid relying on direct translation alone, as nuances and cultural differences in language can distort the sentiment. For example, idiomatic expressions may not carry the same emotional weight in translation, leading to potential misinterpretations.

5. Predictive analytics
Advanced sentiment analysis tools use AI to predict potential escalations based on past patterns. Negative sentiment signals, like frequent complaints or repeated use of terms like “unacceptable,” can flag potential churn risks.
For instance, if you are a SaaS provider, you could use these insights to intervene before customers cancel subscriptions. If predictive models indicate dissatisfaction due to software bugs, you might offer free upgrades or expedited patches, converting dissatisfaction into loyalty.
Two cents from Sprinklr
📈📉 Map sentiment to key business metrics
Sentiment scores should be tied directly to business KPIs, such as net promoter score (NPS), customer retention rates or average response times. This correlation uncovers the “why” behind the numbers.
For example, an online retailer discovers that low NPS scores correlate strongly with negative sentiment about return policies. Armed with this knowledge, they simplify the process, leading to a 25% boost in NPS over the next quarter.
Bonus Read: How to improve the Net Promoter Score of your brand
Top tools for customer sentiment analysis
In today’s crowded market of sentiment analysis tools, it’s essential to prioritize the quality and accuracy of insights over everything else. Here are some of the top tools to consider for analyzing customer sentiment:
Sprinklr Insights
Sprinklr Insights is a comprehensive sentiment analysis platform aggregating actionable insights from over 30+ social and digital channels, 400K+ media sources and 1B+ websites, offering a unified view of customer feedback on a single dashboard.
With advanced AI and machine learning capabilities, Sprinklr Insights captures sentiment across conversations in real-time, identifying key emotions and trends to help you respond quickly and effectively — often, at the moment. By blending text and visual sentiment data, Sprinklr offers a fuller picture of how customers feel about your brand.
Notable features:
- Industry-leading AI
Sprinklr's AI and generative AI models discover trends' root causes and offer clear, actionable recommendations. Achieve an accuracy rate of 90%+ with verticalized AI models and business-specific customizations. Learn more about Sprinklr AI
- Holistic sentiment tracking
Sentiment tracking spans real-time, aspect-based, domain-specific and historical methods to understand customer emotions comprehensively. Together, these approaches ensure a nuanced and actionable understanding of customer attitudes, enabling brands to stay ahead of emerging trends and customer expectations.
- Comprehensive data coverage
A robust sentiment tracking system globally integrates insights from diverse channels — including social media, blogs, news platforms, forums and review sites. Sprinklr allows monitoring across 8+ social channels and hundreds of digital sources, offering an unparalleled view of competitor activities, audience sentiment and market trends, all in real-time.
- AI-powered insights
Advanced AI and natural language processing (NLP) enable sentiment tools to interpret even complex language patterns, such as sarcasm or ambiguous expressions. These nuanced insights clarify how customers perceive your brand, helping you refine your messaging and customer experience strategies.
- Multi-language support
In today’s global marketplace, multi-language sentiment analysis is vital. Sprinklr supports 30+ languages, ensuring accurate sentiment tracking across regions. This particularly benefits multinational organizations seeking to resonate with culturally and linguistically diverse audiences.
- Customizable alerts
Sprinklr’s platform empowers users with real-time, customizable alerts for sudden sentiment shifts. These alerts can highlight spikes in negative sentiment, helping you respond swiftly to potential issues or PR crises. For example, during a service outage, alerts can guide your teams to prioritize customer communication and resolution efforts, minimizing reputational risks.

Brandwatch
Brandwatch offers sentiment analysis, focusing on social listening across various platforms. It captures customer sentiment from online conversations and reviews, providing a dashboard view that categorizes emotions, trends, and shifts in real-time.
Brandwatch also uses AI to analyze text data for brand mentions, detecting emerging trends and sentiments that help businesses stay informed about public perception and respond promptly. The platform emphasizes competitor benchmarking, giving brands a relative view of their performance against others.
Related Read: Brandwatch Alternatives: Top 7 Competitors in 2024
Sprout Social
Sprout Social’s sentiment analysis tool provides brand insights through social media monitoring and customer engagement metrics. By tracking and analyzing customer feedback and interactions, Sprout Social identifies sentiment trends over time, helping businesses understand shifts in customer mood and respond accordingly.
The tool includes a social listening feature that lets brands track specific keywords and phrases. This allows teams to engage with customers proactively and address concerns based on real-time sentiment data.
The Sprinklr Differentiator
While each tool mentioned above offers valuable sentiment insights, Sprinklr stands out with its industry-leading AI and generative AI capabilities, setting a new benchmark in sentiment analysis.
Its AI models go beyond just trend discovery — they dig deeper into root causes, offering personalized and actionable recommendations tailored to your business needs. With a precision rate of over 90%, Sprinklr's sentiment analysis delivers unmatched accuracy, helping businesses confidently make data-driven decisions.
Why Sprinklr?
- Generative AI: Uncovers trends, identifies themes and drills down into root causes with actionable insights.
- Tailored customizations: Business-specific models to suit your unique needs.
- Best-in-class accuracy: 90%+ accuracy with AI models designed for verticals.
Get a Sprinklr Insights demo today and explore how Sprinklr's AI-powered sentiment analysis can drive deeper insights and help you stay ahead of the curve.
Frequently Asked Questions
Businesses can combine quantitative data, like satisfaction scores and response times, with qualitative insights from customer comments to get a full view of sentiment. They can use sentiment analysis tools to categorize qualitative feedback and link it with quantitative metrics for deeper insights.
Challenges in sentiment analysis include language nuances, detecting sarcasm and handling large data volumes. Businesses may also face integration issues with existing systems and accuracy can vary depending on the tool’s capabilities.
Accuracy depends on the quality of the AI model and the data it analyzes. While advanced AI-powered tools can achieve up to 85-90% accuracy, factors like language diversity, slang and industry-specific terminology can affect precision.
Privacy is the biggest concern. Businesses should ensure they have consent before analyzing customer data, store insights securely and avoid overstepping by targeting individuals based on personal emotions. Transparency in how data is used builds trust.
Track metrics like sentiment score, customer satisfaction (CSAT), net promoter score (NPS) and keyword frequency in feedback. These metrics provide a snapshot of overall sentiment and help identify customer satisfaction or dissatisfaction trends.

