The AI-first unified platform for front-office teams
Consolidate listening and insights, social media management, campaign lifecycle management and customer service in one unified platform.
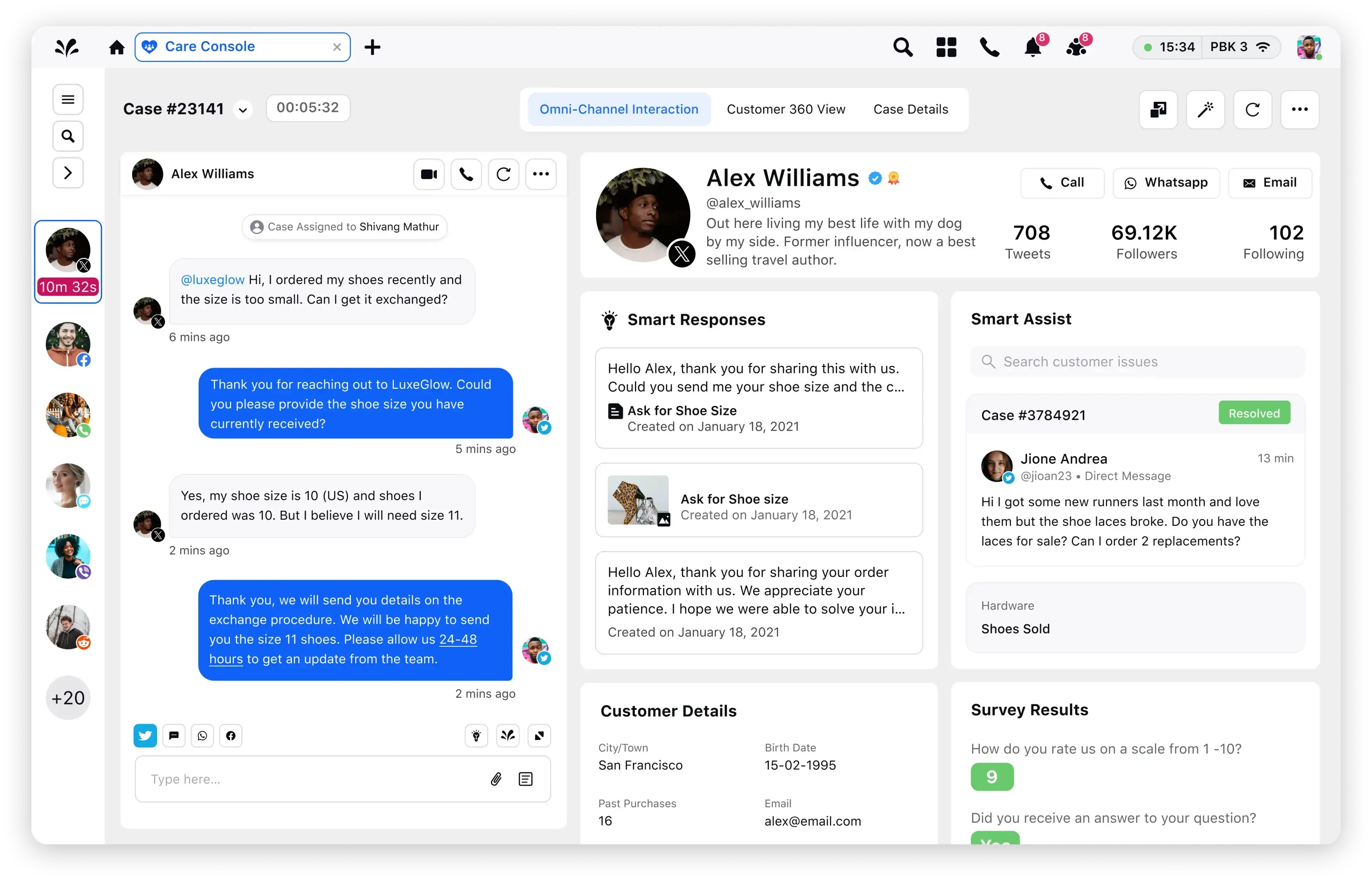
Elevate Customer Interactions with Quicker, More Precise Responses Using Digital Twin’s RAG capabilities
In a world where customer expectations and AI innovations are advancing at a breakneck pace, brands are under immense pressure to adapt and evolve rapidly. As customers' demands more personalized, instantaneous service, the adoption of advanced technologies becomes essential. In this context, Retrieval Augmentation Generation (RAG) stands out as a revolutionary framework for enhancing Large Language Model (LLM) agents to provide unparalleled customer service. These agents leverage their advanced natural language understanding and generation capabilities to enable businesses to offer round-the-clock support, manage complex customer queries, and scale interactions without the constant need for human oversight. A key application of LLM agents includes answering customer questions based on a company’s knowledge base, thereby reducing operational costs while improving the overall customer experience.
This article will delve into how Sprinklr leverages RAG to unlock the full potential of Generative AI for enterprise use cases. You will explore the unique features of Sprinklr’s RAG architecture, its benefits compared to conventional models, and forward-thinking approaches that sets Sprinklr apart in the realm of customer experience and AI-driven innovation.
What is RAG
Traditional customer service models fall short when there is a surge in the volume and complexity of customer inquiries.
Enter RAG. RAG isn’t just another tool. It’s an ally for businesses vying to scale their customer service without a dip in quality. By syncing with databases and knowledge bases, RAG doesn’t just respond; it understands, providing tailored responses that are precise and personal.
The real revolution unfolds at Sprinklr, where we've turbocharged RAG's core abilities to transform customer service from a mere function to an experience. With RAG incorporated into our platform, we've equipped businesses to offer streamlined, dependable solutions to customer concerns while simultaneously slashing operational costs.
Let’s dive deeper into the unique features that make all this possible, setting us distinctly apart in the marketplace.
Sprinklr’s Unique Approach to Using RAG
Sprinklr’s platform not only solidifies RAG as a central component in delivering a sophisticated user experience through advanced knowledge retrieval and optimization, but it also goes beyond RAG by offering forward-thinking capabilities. Let’s explore each of these in detail.
#1 RAG as a central component
- Enterprise-grade RAG architecture:
- The platform boasts a comprehensive collection of connectors to various knowledge management systems, supports the upload of diverse document types, and enables the creation of custom question-answer pairs directly within the user interface. These features collectively foster a robust retrieval augmented generation system
- Effective Training and Indexing:
- Auto-sync of Knowledge Base Content
- Ensures information stays up to date, allowing the user to define the sync frequency as per their choice.
- Optimization:
- Lightning speed indexing of articles; provides a state-of-the-art user experience compared to other bot providers, with less than 2s for time-to-first token.
- Auto-sync of Knowledge Base Content
- Expansion to various file formats:
- Sprinklr bots support images, PDFs with images, tabular and multiple other multi-modal file types, ensuring that any out-of-the-box knowledge content maintained by the brand, is consumable as-is. This ensures that no time or effort is consumed in changing the format of content from the brand's end.
- Document Prioritization:
- Prioritize recently updated content over older ones, in case of any conflicting information in the RAG search results.
- Also supports custom prioritization at the source level.
- Knowledge Optimization:
- The Sprinklr Knowledge Base integration ensures that the data remains unambiguous and of high quality. The platform helps you spot conflicting or duplicate information in your knowledge base. Armed with these insights, you can take steps to fix any inconsistencies. Clearing up this confusion improves your ability to accurately deliver information and responses.
- Golden test set and evaluation:
- In scenarios where 100% predictability of LLM output is unattainable, thorough evaluation across key metrics helps ensure robustness and reliability. A golden test set offers critical insight into the LLM’s behavior, mitigating risks before real-world deployment.
- It helps measure the score across the most relevant metrics:
- Retrieval Precision: This measures the ability of the bot to retrieve relevant information accurately.
- Content Recall: The completeness of the information retrieved by the bot—how much of the relevant information the bot managed to recall.
- Context Retention: It determines how well the bot retains context between consecutive interactions or turns in a conversation.
- Engagement Capability: How engaging and conversational the bot is in maintaining human-like interaction.
- Faithfulness: The degree to which the bot’s outputs are factually correct and faithful to the input data to prevent hallucination.
- Adversarial Guardrail Score: How well the bot handles adversarial inputs designed to confuse, manipulate, or break the system
- Guardrails Library:
- Robust guardrail infrastructure to ensure brand-compliant responses are published to the end user.
- Topic Control:
- Effective management and tagging of knowledge content, allowing brands to narrow down knowledge content to help with user queries.
- Global Control:
- Provides security control on the user's query before it is passed to the LLM by detecting negative sentiments, profanity, or other prompt injection methods including masking PII data.
- Topic Control:
- Robust guardrail infrastructure to ensure brand-compliant responses are published to the end user.
#2 Going Beyond RAG
Now let’s see how Sprinklr goes beyond the basic RAG framework to offer forward-thinking capabilities and further improve responses. These additional features complement RAG and add extra value to its supported use cases.
- Actions:
- Route to agent:
- Knowing when the bot doesn’t know is critical, allowing for timely and appropriate interventions. Smartly decide when to route the user to an agent.
- Fallback:
- Detect when the LLM encounters uncertainty or generates a fallback response and trigger predefined actions.
- Route to agent:
- Streaming responses:
- Stream LLM responses to significantly enhance user experience. We can achieve a sub 2 second first token generation with any state-of-the-art LLMs.
- BYOK/BYOM (Bring your own key/model):
- Sprinklr integrates seamlessly with third-party LLMs, allowing brands full control, if they wish, to use their own LLMs, instead of one provided by Sprinklr.
Details of Experiments:
All the experiments were done from US-east-1 region.
File Formats Supported:
Sprinklr: pdf, (with multimodality support), html, xml, xslt, plain text, csv, xls, xlsx, json, rtf, ppt, pptx, docx, txt, jpg, jpeg, png
Assistants API: c, cs, cpp, doc, docx, html, java, json, md, pdf, php, pptx, py, rb, tex, txt, css, js, sh, ts
Cohere RAG: pdf, txt
AmazonQ: pdf, html, xml, xslt, md, csv, xls, xlsx, json, rtf, ppt, pptx, docx, txt
Summary
In conclusion, Sprinklr’s RAG capabilities offer a transformative approach to customer interactions, blending speed, accuracy, and scalability. Sprinklr delivers a seamless user experience by integrating enterprise-grade RAG architecture with powerful features. The platform’s focus on continuous knowledge optimization and robust guardrails ensures that brands can maintain high-quality, compliant, and contextually relevant responses. This makes it a standout solution for enterprises aiming to elevate their customer service.
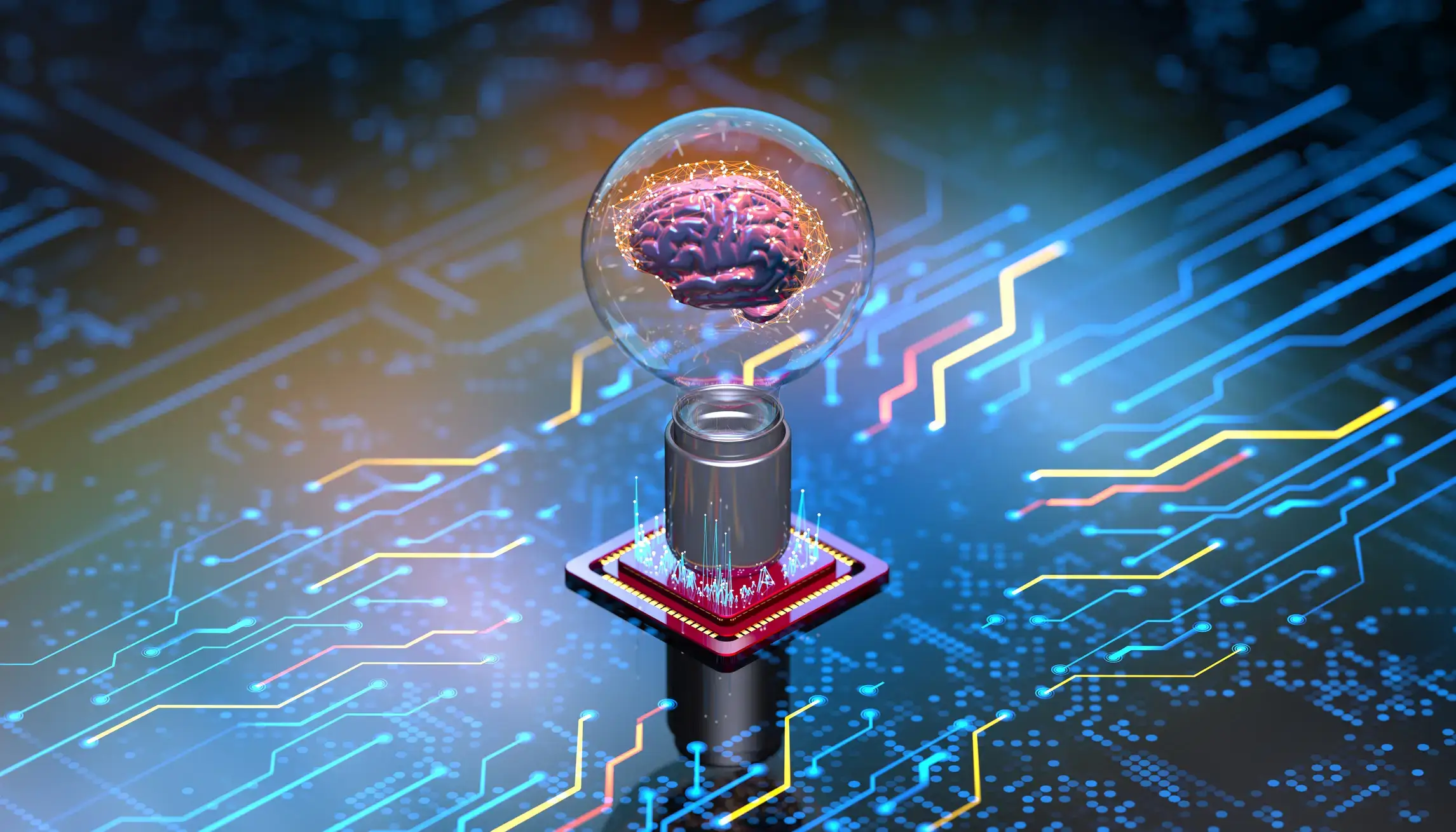
