The AI-first unified platform for front-office teams
Consolidate listening and insights, social media management, campaign lifecycle management and customer service in one unified platform.
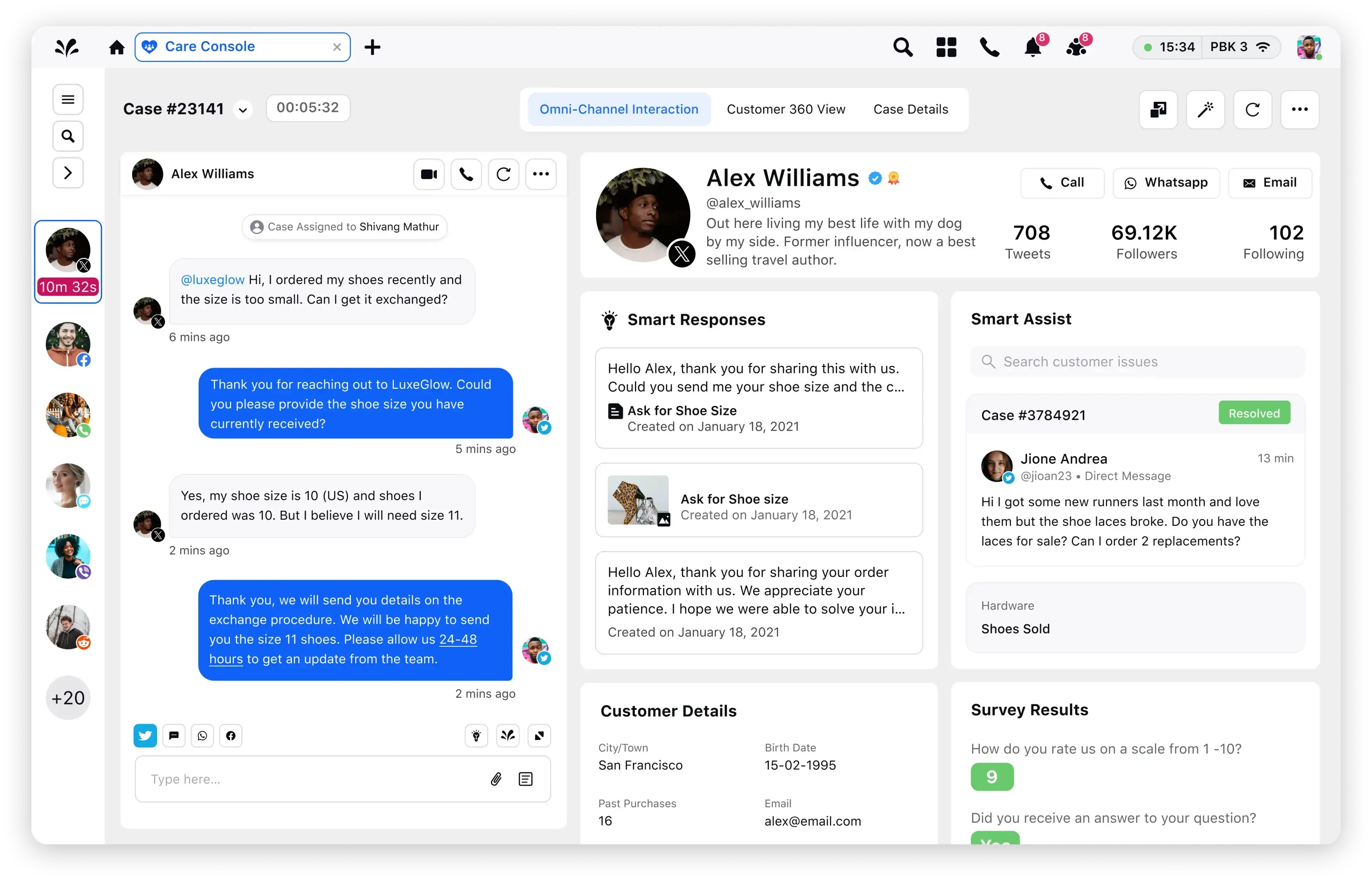
How AI powers Revenue Generation in Contact Centres
In this article, CX Thought Leader Martin Hill-Wilson draws our attention to McKinsey's customer care survey that highlights a growing focus on revenue generation in contact centres, with 20-25% of positions now directly contributing to sales. Equipped with real-time analytics this 'Sales through Service' strategy empowers advisors with AI-driven insights for tailored, timely offers, potentially transforming contact centres into proactive revenue drivers.
The most recent version of McKinsey’s annual State of Customer Care survey reveals an interesting trend. The consistent rise of interest in revenue generation within contact centres.
CX and Service Industry analyst Nicolas de Kouchkovsky picked this out in a LinkedIn article, noting how “revenue generation skyrocketed from less than 5% in 2016 to over a third of respondents rating it a top priority in 2023.”. He and industry experts estimate between 20-25% of contact centre positions now play a direct role in generating revenue.
Whatever the actual number, the motivation behind it makes sense. Moving from cost towards profit centre status adds internal kudos and negotiating strength for service leaders. It also appeals to customers when offered better solutions, more features, or added value that meet their needs more effectively.
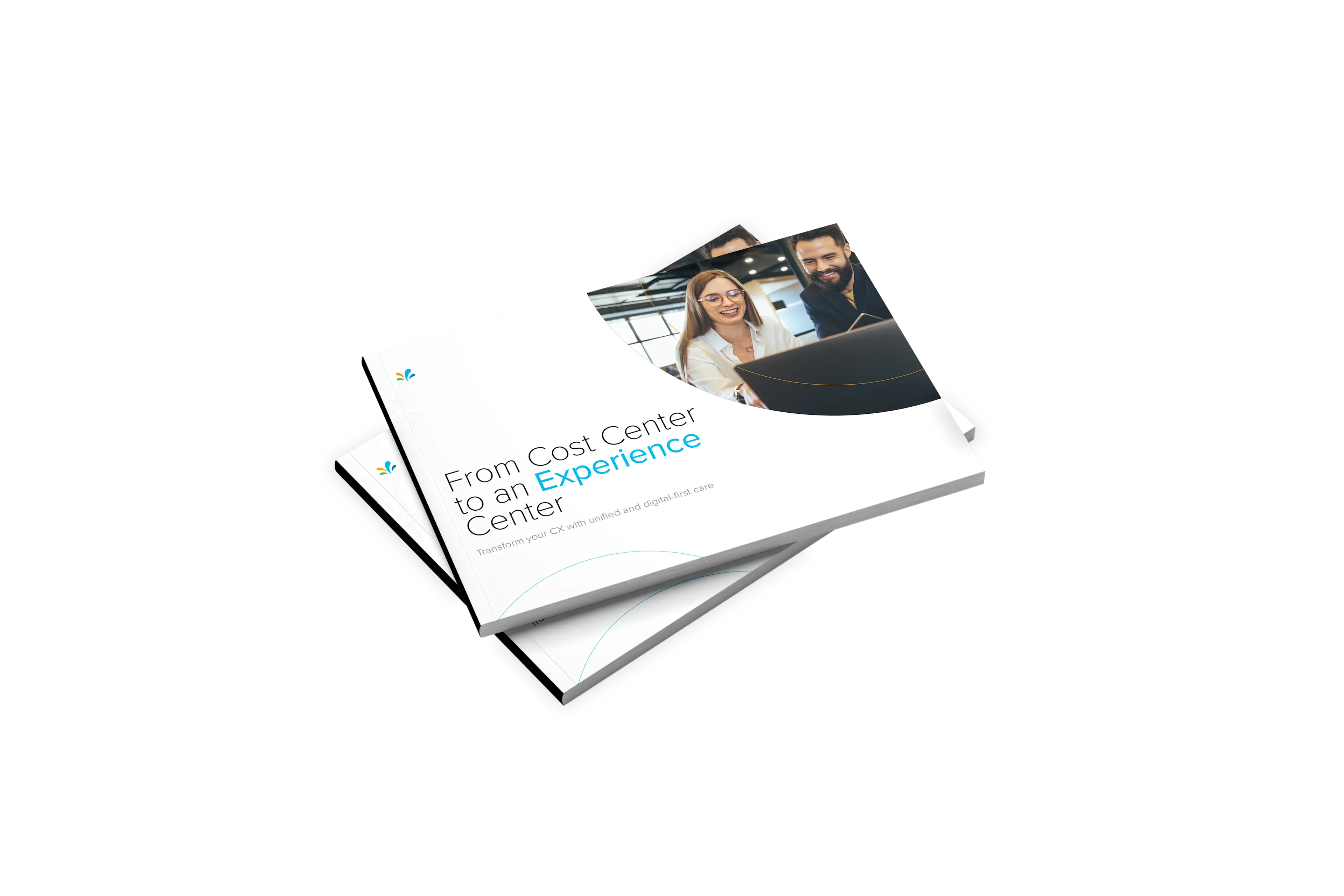
Listening in to typical customer support interactions explains why and how it works. Attentive advisors use the rich flow of insight about customer preferences and pain points to sense when the moment is right to steer a service conversation into a sales opportunity.
Yet ‘Sales Through Service’ is nothing new in many sectors.
As a smartphone user, you are likely to have experienced this for yourself. You call in or message with a query about your data plan. After resolving your query, the advisor informs you about a higher data plan that offers better value based on your usage patterns. You agree and upgrade.
If ‘Sales through Service’ is customary practice in some sectors, McKinsey’s research shows more organisations are continuing to make revenue generation a strategic customer care priority.
So, should you? And if you already have, how much better could you become?
This is a timely question to ask in today’s world of real-time, AI powered conversation analytics. Can AI models become trained to spot what an experienced advisor can intuitively sense? If so, then these models offer many opportunities to scale your Sales through Service strategy. First by empowering a broader cohort of advisors. Then by delighting customers with relevant, personalised recommendations.
Please note within the context of this article, ‘real-time analytics’ is shorthand for conversation analytics which includes voice, text, and video. Also, distinctions between speech and voice analytics are ignored given the speed at which AI models continue to redefine their capabilities.
The Art of The Possible
Let’s explore the art of the possible in this rapidly evolving capability by creating a North Star for you to form your own Sales through Service strategy around. Hopefully as an inspirational outcome of reading this article.
Our exploration starts with a foundation understanding of the enabling technologies that power real-time analytics. As context, we’ve just gone through a decade of rapid evolution. This continues to be driven by a convergence of cloud driven computational power, improved quality and quantity of data and technical breakthroughs in the design of algorithms and the AI models they generate.
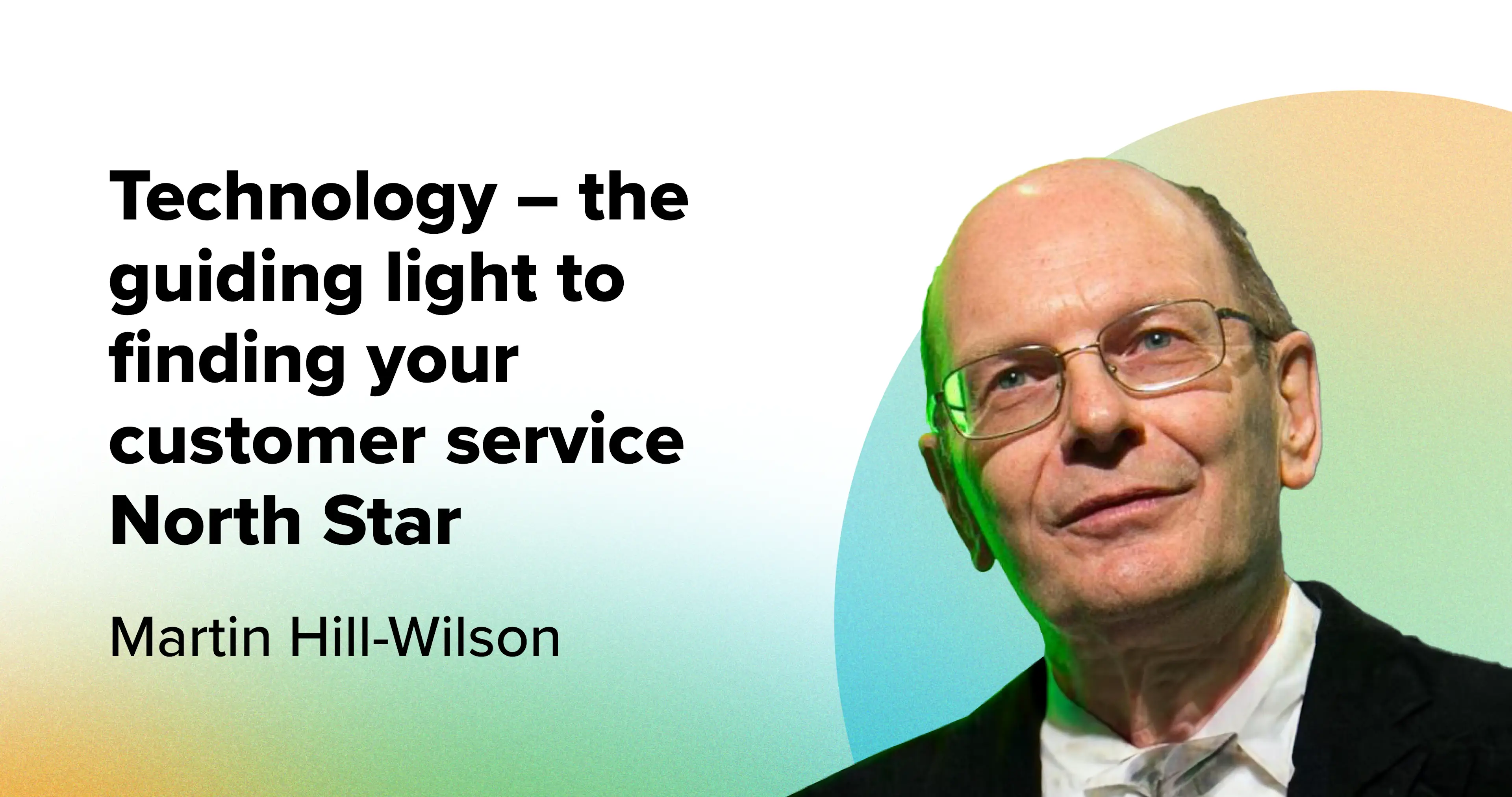
The combined impact is that these enabling technologies are now faster, more capable, and easier to use. More specifically, Machine Learning, Deep Learning, Natural Language and Generative AI allow us to reimagine how the full lifecycle of customer engagement is designed, managed, and optimised. And within that ambition, how contact centres become a revenue generating touchpoint.
This is a big idea. We know such transformations are multi-year change programmes. Yet with agile planning and deployment, they can still yield short term benefits. For instance, real time analytics can be deployed rapidly with an immediate impact on revenue generation.
Over time, performance levels will improve as more capability is deployed. Two obvious examples are to upskill more customer facing teams in consultative selling and integrate additional sources of customer data such as purchase history to improve the predictive accuracy and recommendations that the AI models driving real-time analytics can make.
Best Practice in Cross Selling and Upselling
But what do ‘faster and more capable’ AI models really mean in the context of spotting revenue generating opportunities during a service conversation?
‘Faster’ translates into real-time responsiveness. In terms of the art of the possible it is now possible to understand all customer conversations as they take place and therefore influence outcomes.
To better understand what ‘more capable’ means let’s look at some well-known best practices and challenges in cross selling and up-selling. Then explore where latest generation AI models add value.
Active Listening and Personalization
By actively listening to customer concerns and preferences, advisors can match their upselling suggestions to align with the customer's specific needs, making the offer more relevant and appealing.
Equally it is just as important to recognise situations in which a customer has done their research and knows exactly what they want. In which case, pushing additional or higher-end products is more likely to lose goodwill and trust.
Educating Customers
Often, customers are not aware of the additional features or higher-tier products available. Advisors can educate them about these options, highlighting the benefits in a non-intrusive manner.
However, if it’s evident that the customer has budget constraints, suggesting more expensive options or additional products might not be well-received and could make the customer uncomfortable. Also, advisors lacking in depth product knowledge can expose their organisations to the consequences of mis-selling.
Timing is Key
Identifying the right moment during a support call is crucial to steer the conversation in a new direction. The best time is often after successfully resolving a customer’s issue when their satisfaction with the service is high. In contrast, attempts to upsell or cross-sell customers while still in the mindset of fixing a problem or complaining about an issue will impact them as insensitive and unwelcome distractions.
Equally during personal moments of heightened anxiety such as a pandemic, cost of living or bereavement, upselling and cross-selling can be experienced as opportunistic or insensitive.
How Analytics Supports Best Practice in Revenue Generation
With these best practices and challenges now front of mind, let’s return to the question of how ‘more capable’ AI models can help.
If there is a core characteristic in the type of AI models we’ve been discussing, it is their ability to surface patterns in conversation data.
These could be recognising different topics and conversational flows between customers and advisors. The mood of those conversations to enable greater empathy. Their needs and preferences to tailor recommendations. Buying signals, inferred from keywords, phrases and language characteristics such as tone, pitch, stress levels or intensity of engagement. Or the behaviours and language used that contrast highest and lowest performing advisors.
This is what speech and text analytics began tracking over two decades ago. Albeit with less sophistication and accuracy. Fast forward into the slipstream of current capabilities and today’s AI models can match complex patterns in the topics, sentiment, and situational context of an individual customer conversation against any reference patterns those models were pre-trained to recognise. All in real time.
The reference pattern could a recurring behaviour such as when customers make contact about their data plan, the majority agree to upgrade. Therefore, whenever this matches the pattern of a live conversation, relevant recommendations automatically appear on an advisor’s screen then iterate based on the ongoing flow of conversation.
Put another way, the Natural Language model that was listening in real time activates a recommendation model which is pretrained on cross-sell/upsell combinations and associated incentives. Also activated is a model able to generate onscreen scripts and nudges then call up workflow to steer that service conversation into a sales conversation and hopefully a processed order.
In Conclusion
This is the value of real-time analytics today. It is already much improved in terms of accuracy and ability to make sense of topics and conversational mood. Its current trajectory suggests we can keep expecting better performance levels for the immediate future.
However contextual awareness remains an ongoing challenge even in the latest Generative AI models. Setting realistic stakeholder expectations is therefore important. Also brief and train front line teams on the value of human judgement and when to use it in relation to the automated recommendations they will receive on screen.
Learning from instances of inappropriate recommendations to improve model performance is another task that will need operationalising. This need for strategy will remain for the near future as our exploration of best practices and associated challenges indicated.
As a North Star, real time conversation analytics offers transformational benefits.
- It helps you identify those conversations most likely to become up-sell or cross-sell opportunities.
- It accelerates the path to sales competency and confidence in a contact centre world often challenged by remote work, ongoing attrition, and widespread reluctance to ‘become a salesperson.’
- Contact centres can evolve into integrated service and revenue hubs which raises their internal status and enriches career path options for service trained teams.
- The behaviours and mindsets of best performing advisors are more quickly discovered, shared, and embedded. With the additional benefit of reduced effort for operational management given the advanced levels of automation designed into the latest solutions.
- Live feedback on every customer conversation also becomes a viable milestone: accelerating self-learning and powering individual paths to competency.
- Genuine opportunities to delight customers with relevant recommendations will now occur more often. It’s worth nothing that when clear cut instances of CX ROI can be hard to find, revenue generation from ‘sales through service’ becomes a shining example.
I’m quite sure that real time analytics will only accelerate the McKinsey trend mentioned at the outset. It will lower the bar to entry for those yet to make the leap. And will boost existing revenue generation programmes by improving advisor productivity and opportunity discovery.
I hope you are inspired to craft your own North Star and benefit from real time analytics.
Interested in discovering additional insights about AI's role in customer experience? Listen to the latest episode of the CX-Wise podcast, where Howard Tiersky, the CEO and founder of FROM, an award-winning digital transformation agency, shares his approach to leveraging AI for refining and streamlining customer interactions.
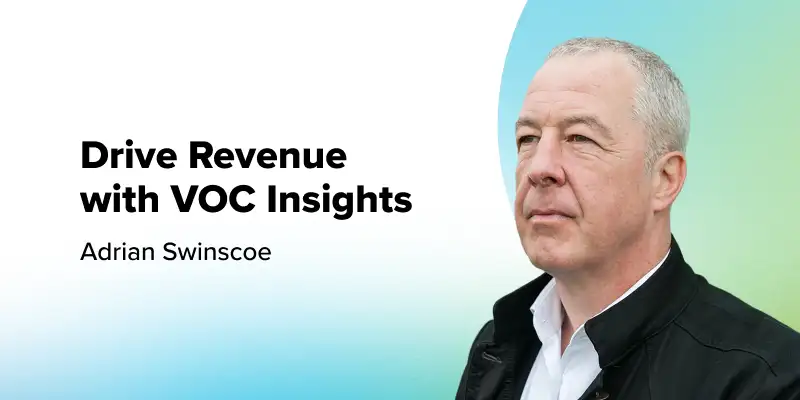