- What is conversation analytics?
- Why is analyzing customer conversation important?
- Conversational analytics vs. traditional analytics
- 5 benefits of conversation analytics
- How does conversation analytics work?
- How to implement conversation analytics [+Strategy]
- Examples of businesses using conversational analytics
What is conversation analytics?
Conversation analytics is the process of assessing customers’ conversations to gather insights about their behavior. The aim is to leverage this intel to personalize future conversations, improving both customer satisfaction and engagement.
From a contact center perspective, using conversation analytics is the best way to collect unsolicited feedback about the business — allowing you to read and analyze your customers’ intent, emotions and actions.
Learn more: Why Conversational Analytics Is the Missing Piece in CX
Why is analyzing customer conversation important?
Analyzing customer conversations is important because it gives you the full context. It reveals the full story, as over 50% of customers use phone support to connect with contact centers. It, in turn, enables real-time conversation analytics to reveal in-depth insights.
It captures both spoken and unspoken cues, going beyond brevity and cognitive load and combines various feedback types to deliver a holistic understanding of a customer’s intent and sentiment.
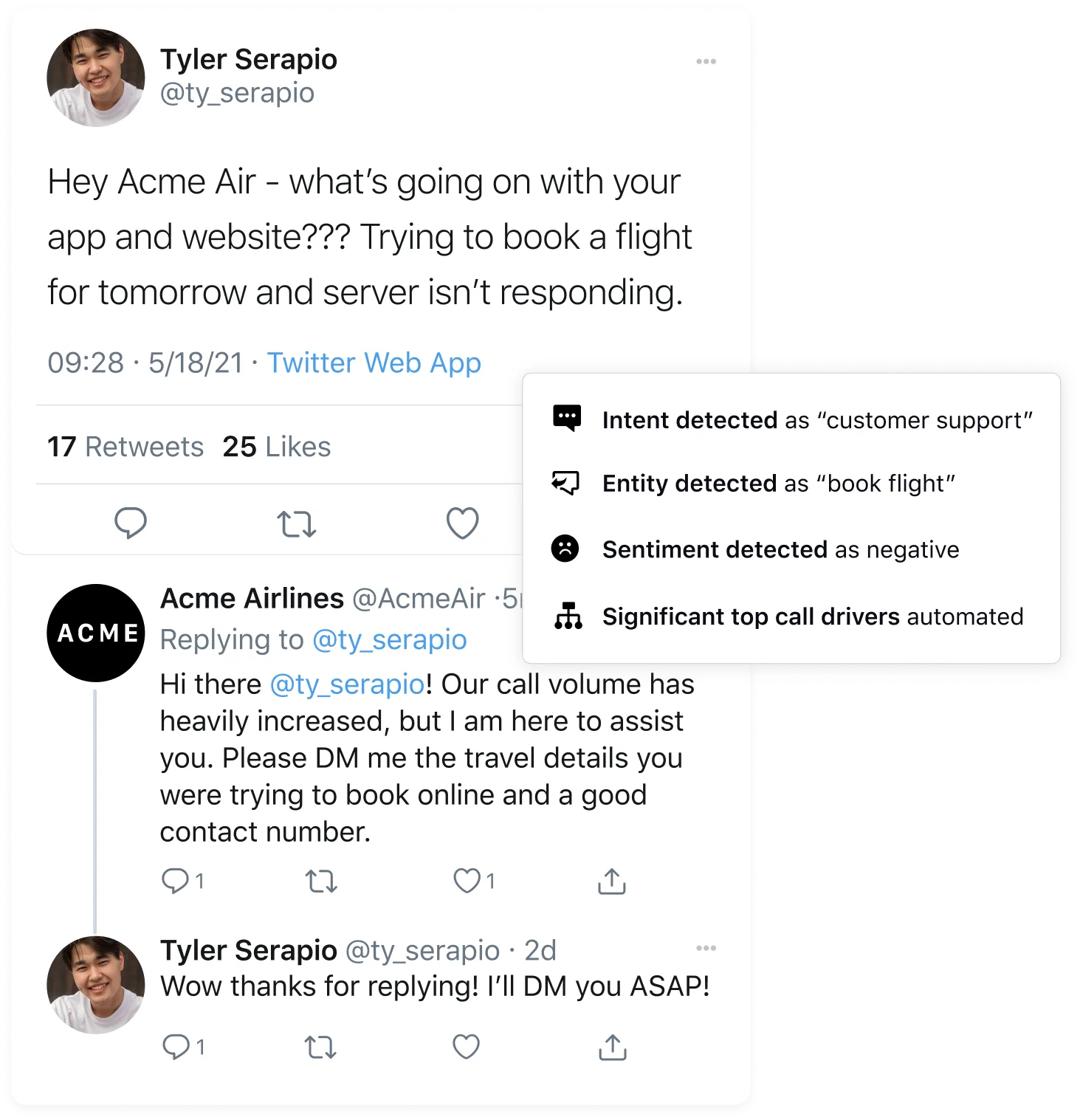
The real beauty is that it enhances the customer journey without burdening users with too many questions, drawing insights from their own initiated conversations, and ultimately preserving the customer experience.
Traditional analytics gives you data in bits and pieces, but conversational analytics helps you discover immediate actionable opportunities to make customer service better. Let’s look at the finer differences between the two below.
Conversational analytics vs. traditional analytics
Conversational analytics is a game-changer for contact centers, as it focuses on real-time insights. Traditional analytics, on the other hand, offers broader business insights but may not be as agile in addressing customer needs during live interactions. Here is a comparison between them: -
Aspect | Conversational analytics | Traditional analytics |
Data source | Conversations and interactions between agents and customers, typically on voice, chat, email and social media | Historical data, often structured, from various sources, including customer databases, CRM systems and call records |
Scope | Focused on the analysis of real-time customer-agent interactions | Broad analysis of historical data to gain insights into overall business operations |
Real-time | Yes, enables immediate intervention and improvements | Primarily retrospective; does not offer real-time monitoring or intervention. |
Metrics | Revenue, customer churn, conversion rates and overall business efficiency | |
Decision making | Governs decisions around customer service quality and agent training | Enables marketing, sales and customer support to make data-driven decisions |
Use cases | Used to assess customer service quality, customer sentiment and agent performance | Used to evaluate website performance, e-commerce sales and marketing campaign impact |
Technology | Relies on AI, natural language processing (NLP), and machine learning to process unstructured conversational data | Typically relies on structured data analysis tools, reporting software and historical databases |
Both have their place in contact center operations, with conversation analytics emerging as a critical tool in today's customer-centric world.
5 benefits of conversation analytics
Listening to your customers and understanding what they say is key to your business’s success. And when you do it precisely leveraging conversation analytics, you reap these five benefits.
Benefit #1: Time savings
Consider a scenario where you need customer feedback for an upcoming product launch. Traditionally, this involved sending out surveys, waiting for responses and manually aggregating data – a process that could take weeks. With conversation analytics, you can collect feedback in real time during customer interactions.
Example: A customer chats with your support agent, expresses their thoughts on the product, and the analytics system automatically categorizes this feedback without disrupting the chat. This saves time and allows you to gather insights on the fly, expediting decision-making and product enhancements.
Benefit #2: Empowered agents
Imagine a contact center agent using conversation analytics. As they engage with customers, the system provides real-time insights into each customer's preferences, previous interactions and concerns. provides real-time insights into each customer's preferences, previous interactions, and concerns.
Example: During a call, the system detects that a customer has had recent issues with the product. The agent can then tailor their conversation, addressing the concerns and suggesting solutions based on the customer's history. Empowered with a 360° customer view, agents are in a better position to provide personalized support and improve customer experiences in the contact center.
Benefit #3: Accurate, high-quality data
Traditionally, gathering customer feedback often involves customers self-reporting their experiences, which can be biased or incomplete. In contrast, conversation analytics relies on technologies like Natural Language Processing (NLP), AI and Machine Learning (ML) to analyze data objectively.
Example: When a customer provides feedback in an open-text format, the system uses NLP to analyze the text, categorize sentiments and extract valuable insights without human interpretation. This data-driven approach ensures the analysis is precise and free from subjective bias, providing businesses with more reliable and accurate information to make informed decisions.
Interesting read: A Comprehensive Guide on Sentiment Analysis
Benefit #4: Democratized data
For global brands that collect customer feedback across various languages and regions, AI-powered conversation analytics can be a boon. Using it, data can be interpreted and understood regardless of language or location.
Example: A sales manager in the US can access insights from customer conversations in Asian languages without prior experience in analytics tools. Data democratization ensures that insights are accessible to everyone, breaking down language and geographical barriers and making data-driven decisions possible across the organization.
Benefit #5: Compliance and risk mitigation
There are situations where a contact center's agent inadvertently breaches compliance regulations during a call. Real-time monitoring identifies this immediately, allowing the issue to be resolved and mitigated before it escalates, resulting in regulatory penalties.
Suggested read: A Contact Center Compliance Guide + Checklist
How does conversation analytics work?
Conversational analytics collects and analyzes communication across various customer service channels such as chat, email, social media, voice and more. Here is a brief overview of how conversational analytics works:
🔎 Data cleanup
After collecting data, AI cleans it up. It removes things like unnecessary words, punctuation and special characters. It ensures everything looks consistent in terms of spelling, grammar and writing.
📖 Understanding language
AI leverages Natural Language Processing (NLP) to break down the text. NLP helps you figure out what words, phrases and sentences mean, considering the context.
📲 Finding patterns
Then, Machine Learning (ML) is deployed to spot trends and patterns in the data, such as recurring issues and customer preferences, using keyword spotting.
💡 Reporting insights
Finally, AI presents the findings in digestible formats like dashboards and reports, pointing out areas of improvement at agent and team levels and how to make customers happier.
How to implement conversation analytics [+Strategy]
Conversation analytics is incredible and what it can do for your business looks great on paper, but a lot depends on how you implement and execute it. Here’s a step-by-step strategy to successfully integrate conversation analytics into your contact center operations.
#1. Define objectives and scope
Outline the objectives for implementing conversation analytics. Determine what you aim to achieve, such as improving customer service, optimizing agent performance or enhancing customer insights. Specify the scope, including the channels (phone, chat, email) to be covered.
#2. Choose the right analytics platform
Select a conversation analytics platform that aligns with your customer service objectives and scope. Ensure it offers the necessary features like real-time monitoring and the ability to process data from various channels.
💡 Expert Tips for Tool Selection
The tool should seamlessly integrate with your existing CRM and tech stack. CRM Integration enables a 360-degree view of customer information and allows for more personalized and effective interactions.
Security and compliance, such as GDPR or HIPAA, should be a focal area in the vendor terms and conditions. A publicly available trust center is a must.
Your best bet is inbound contact center software that uses ML models specifically trained on diverse accents, dialects and industry-specific jargon. This way, you can cater to a global audience seamlessly.
#3. Data collection & pre-processing
Gather a sample of customer interaction data from the selected channels. Ensure the data is well-structured, covering a sufficient time period. Clean and pre-process the collected data by removing noise and irrelevant information and ensuring consistent formatting.
#4. Speech-to-text conversion (if applicable)
Convert voice interactions to text using speech-to-text technology if you plan to analyze voice calls. It is made possible through Automatic Speech Recognition (ASR). ASR, armed with algorithms trained on extensive audio data, excels in recognizing speech patterns and converting spoken language into written text. Its role is indispensable in making voice data accessible and searchable.
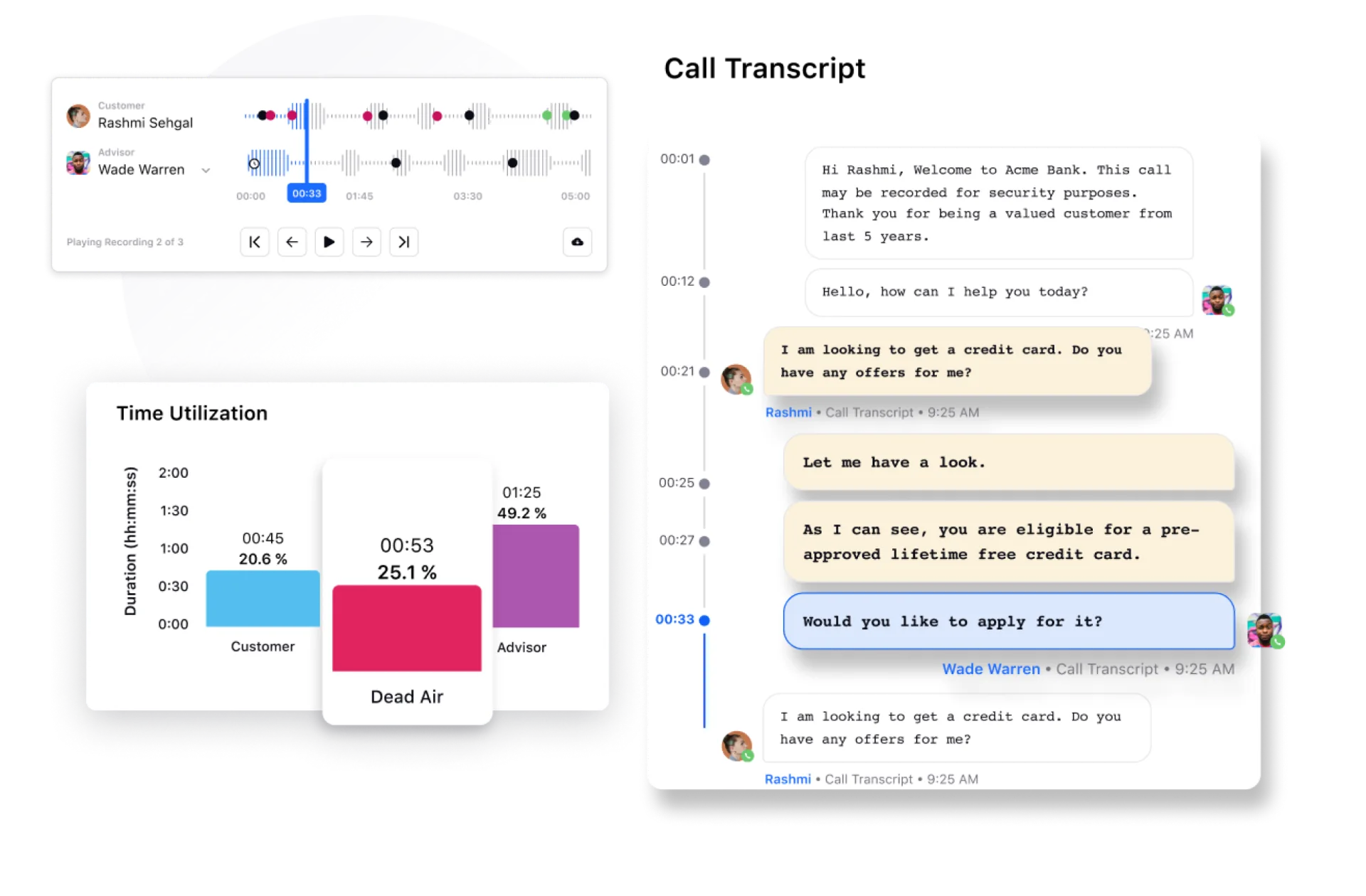
Conversely, leverage Text-to-Speech (TTS), which acts as the vocal communicator. TTS takes the written text and breathes life into it, a vital tool when systems like voice bots need to engage users vocally, ensuring seamless communication and enhancing the customer experience.
#5. Sentiment analysis
Apply sentiment analysis to gauge customer sentiment in the conversations. Categorize sentiment as positive, negative or neutral.
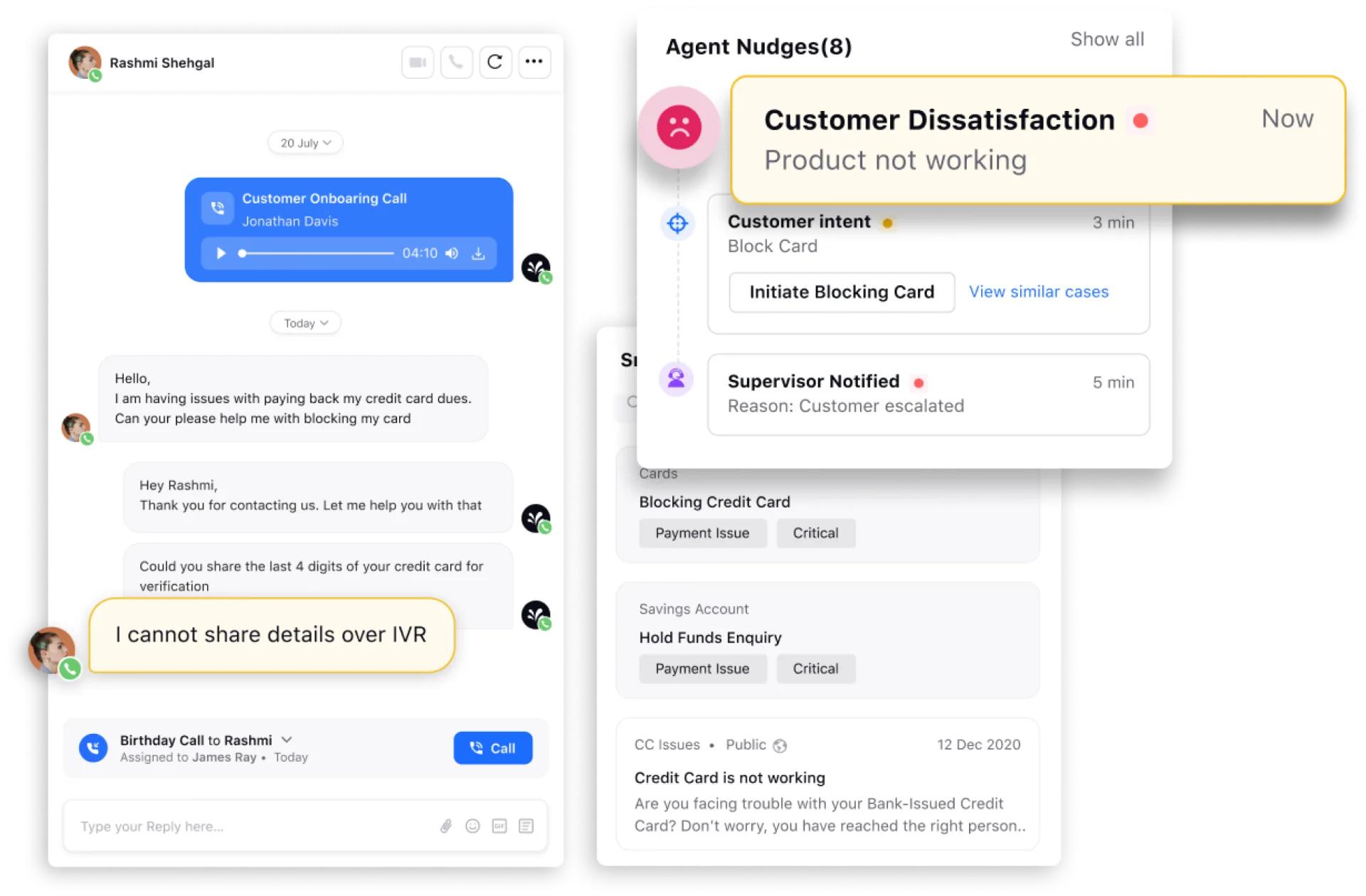
To accomplish this, employ a sophisticated technique that employs machine learning, statistics and NLP to unearth the spoken and unspoken customer emotions in interactions. There are several key types of algorithms that power sentiment analysis models.
Rule-based approach: It defines polarized words as positive, negative or neutral in the text. It uses NLP techniques to create customized rules that help the system count polarized words and identify sentiments.
Automatic or machine learning-based sentiment analysis: Machine learning models are trained using datasets that contain positive and negative words. Once the training is complete, these models can automatically detect the tone or sentiment behind the text.
Hybrid sentiment analysis: It combines both manual and automatic approaches for sentiment analysis. This approach is renowned for its ability to produce highly accurate results.
#6. Real-time monitoring
Implement real-time monitoring to detect issues as they occur. This enables immediate agent interventions. These insights are not confined to one format or one device; they can be effortlessly shared across your entire organization in various formats and on all devices.
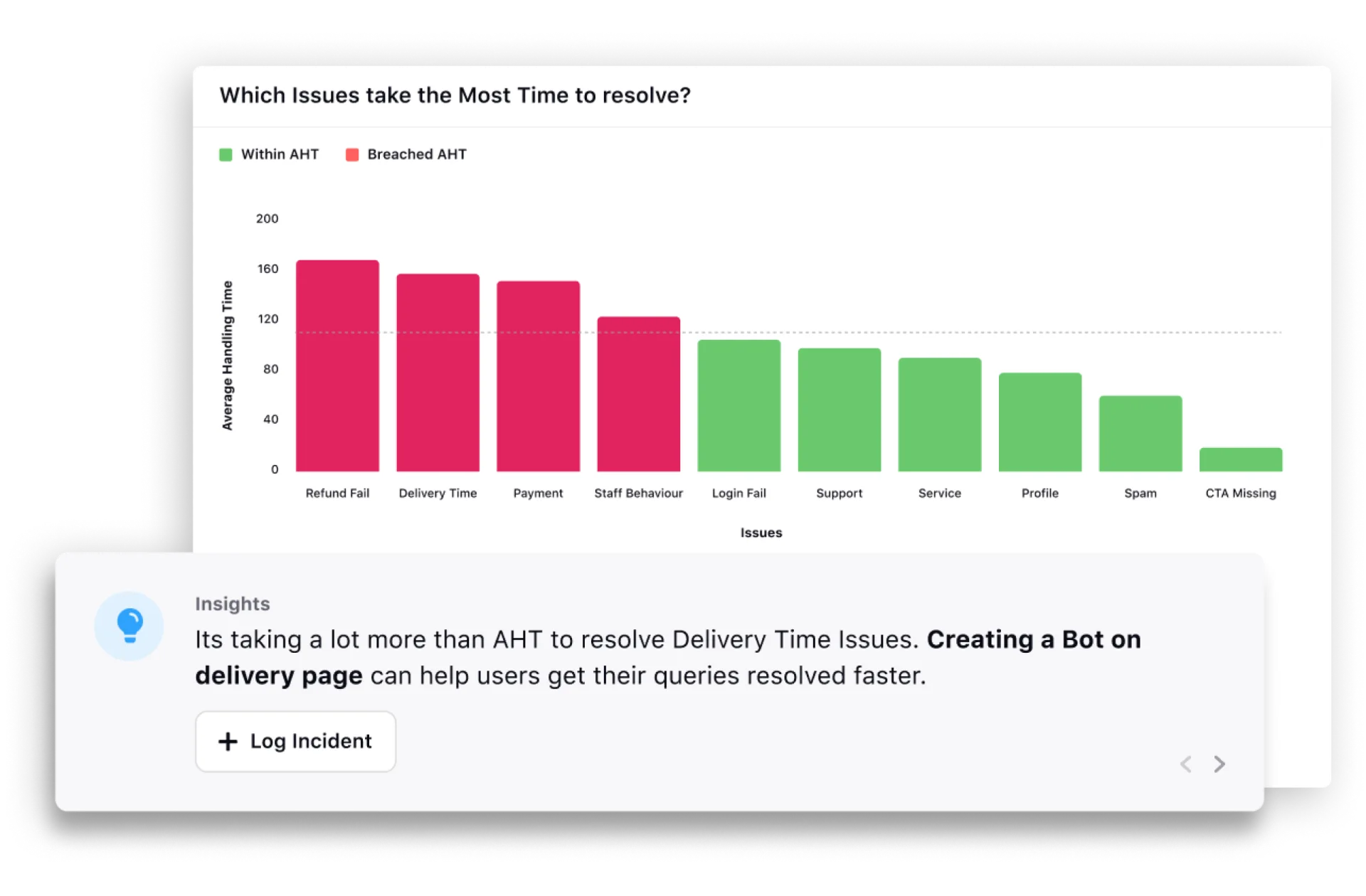
#7. Agent performance analysis
Evaluate agent performance during the interactions using agent performance metrics such as response time, script adherence, AHT and issue resolution. These data points can be visualized through dashboards and reports. You can get a complete view of how your agents perform daily, monthly and quarterly.
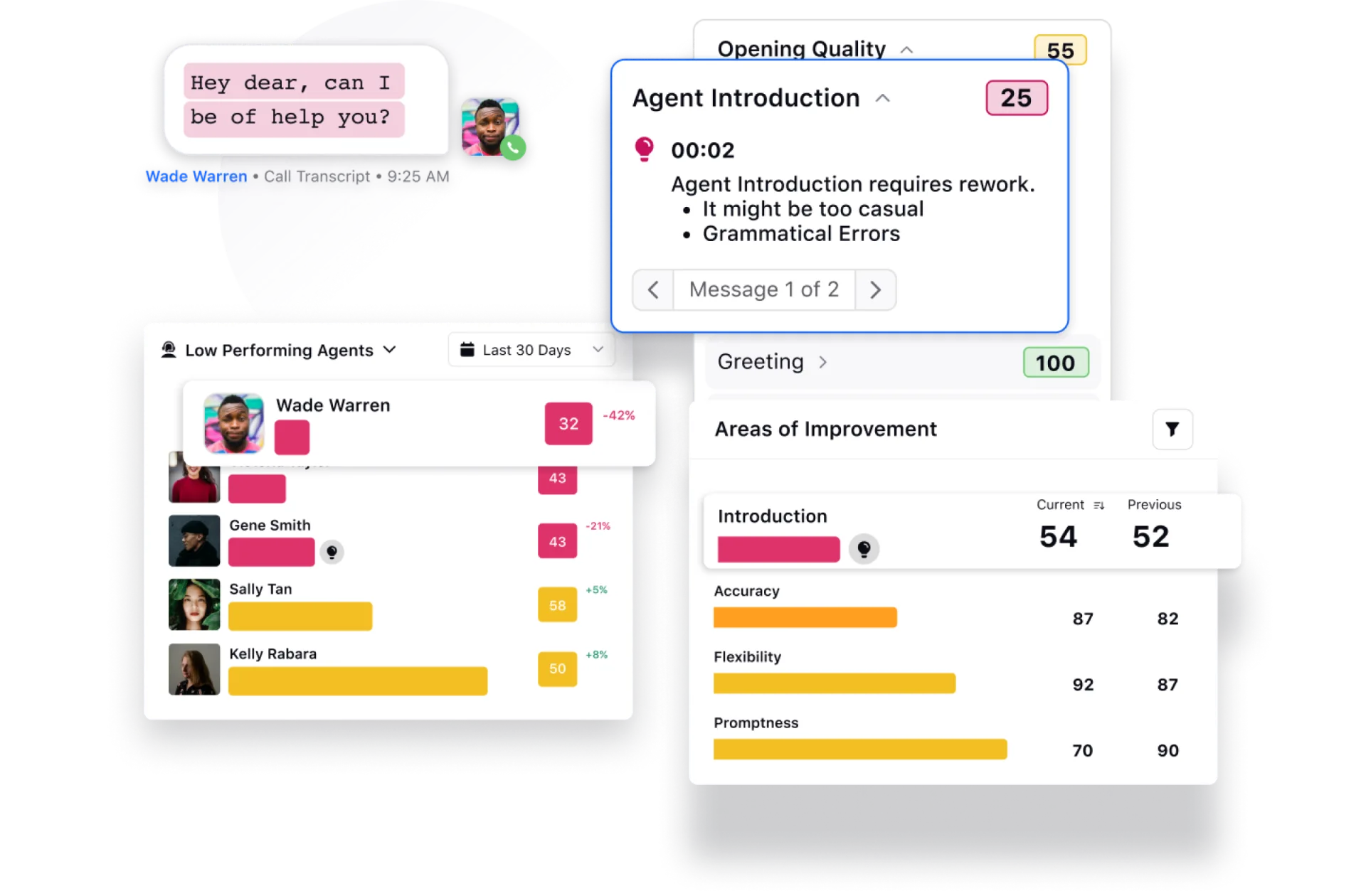
#8. Customer insights
Gain insights into customer preferences and pain points. Identify recurring issues and opportunities for proactive support through data points projected on dashboards and reports.
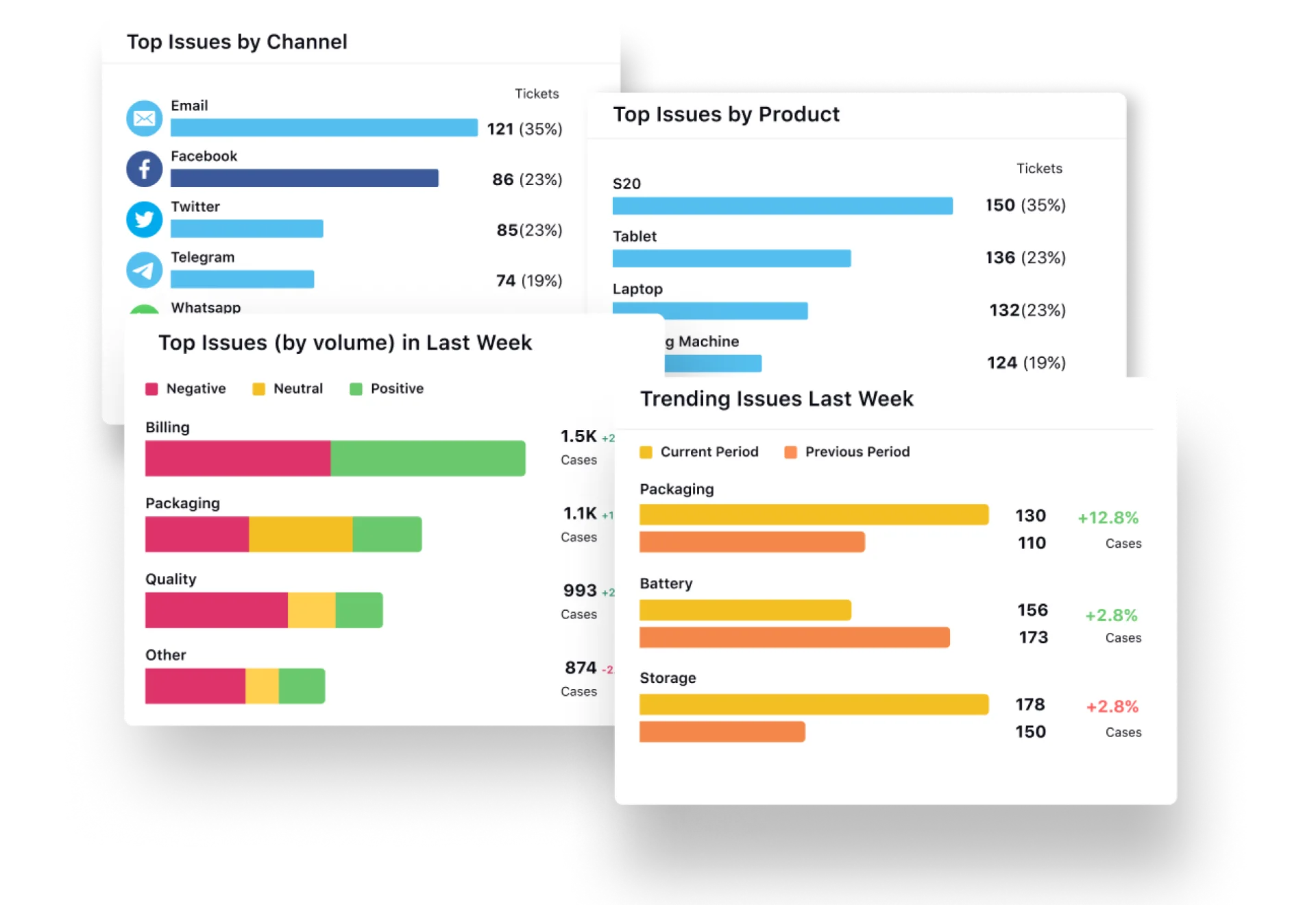
#9. Compliance and data security
Ensure the implementation complies with data privacy and security regulations, maintaining customer data integrity.
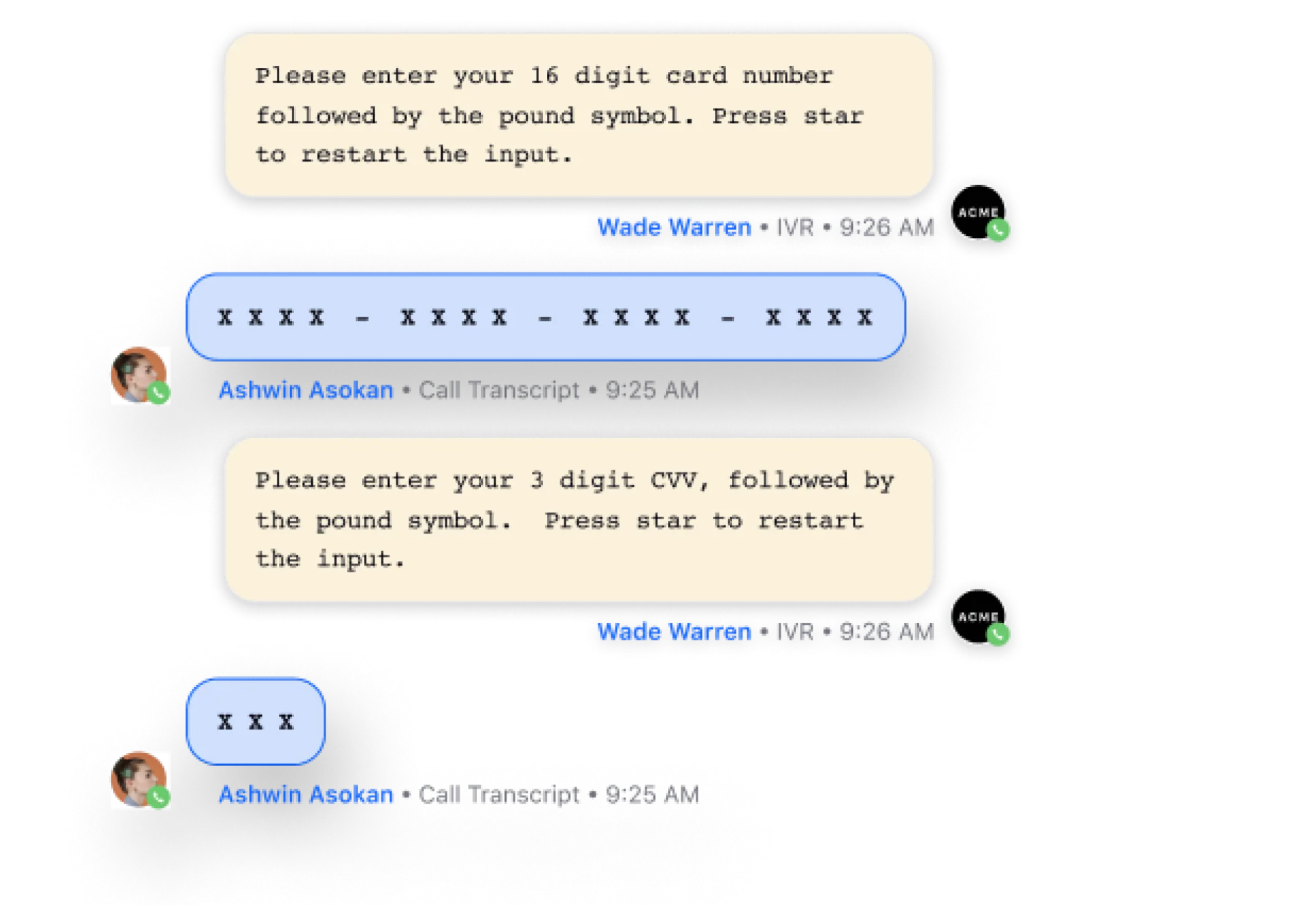
Examples of businesses using conversational analytics

When Intuit QuickBooks introduced its chat experience, it faced the challenge of analyzing weeks of unstructured data. To swiftly address customer concerns and reduce escalations, they adopted conversational analytics. This platform provided full conversation insights and flow mapping.
The result?
Decrease in fallbacks and a remarkable reduction in human escalations. Speed and insights made all the difference.

Cdiscount, one of the top French e-commerce companies, faced heavy traffic of inbound customer inquiries – four million calls, 300,000 social media conversations, and millions of messages between customers and sellers every year. Cdiscount wanted to gain a comprehensive understanding of how customers perceive their brand.
The challenge: Due to the sheer volume of customer contacts and the diversity of communication channels used, traditional analysis methods were useless. They needed to find a solution that was capable of extracting meaningful insights from the vast expanse of customer interactions.
The solution: Cdiscount deployed Sprinklr Service, which enabled them to analyze customer interactions from various channels and act on insights to boost customer relationships and the overall experience.
Utilizing Sprinklr AI, they were able to:
Transcribe and analyze voice, chat and social interactions.
Pinpoint conversation themes like delivery issues and refunds.
Extract reports on trends in customer conversations and satisfaction drivers.
The result: Cdiscount now analyzes 100% of customer support calls and over 75,000 conversations. Agents receive quality and CSAT scores for interactions, with CSAT scores increasing by 15%.
Now, every interaction gets a quality score, and all interactions receive a CSAT score that has surged by 15% since the launch. This has significantly improved the quality of agent-customer interactions. Read the full story here>>
“Sprinklr provides us with a unique platform that allows us to improve each customer experience while giving our agents the opportunity to become top performers.”
Louis Brun-Ney
If you want to replicate Cdiscount’s success and create better customer experiences, take a free trial of Sprinklr Service for 30 days and let us know how you find it.
Frequently Asked Questions
Thank you for contacting us.
A Sprinklr representative will be in touch with you shortly.
Contact us today, and we'll create a customized proposal that addresses your unique business needs.
Request a Demo
Welcome Back,
No need to fill out any forms — you're all set.