- What is customer analytics?
- 6 Steps to analyze data for customer analytics
- Categories of customer analytics
- Types of customer analytics
- Why do businesses need customer analytics?
- Key metrics associated with customer analytics
- Industry-specific use cases of customer analytics
- The role of AI in customer analytics
What is customer analytics?
Customer analytics, or customer data analytics, involves gathering, analyzing and interpreting data about your customers' interactions with your brand across various touchpoints.
This approach goes beyond basic metrics by delving into behavioral patterns, preferences and motivations. Customer analytics provides a holistic view of your customer journey by leveraging data from sources like website visits, live chats, customer calls, surveys and even social media interactions.
Unlike metrics, which offer snapshots — such as the total number of purchases or a bounce rate—customer analytics helps you understand the "why" behind the numbers. For instance, while a metric might show that a product isn't selling well, customer analytics could uncover customers abandoning their carts due to a complex checkout process.
While customer analytics lets you analyze real-time data, predict customer behaviors and deliver highly personalized experiences that drive customer loyalty and business growth, behind the scenes, various stakeholders work together to make customer analytics work — data scientists, marketing teams, customer service heads, product managers and CX strategists. Their collective expertise ensures that you continually interpret data and act on insights to enhance the customer experience.
6 Steps to analyze data for customer analytics
It would be best to have a structured implementation plan to get the best out of customer analytics. Here are the steps to follow:
Step 1: Define clear goals
The foundation of any customer analytics strategy is a well-defined set of objectives. Applying the SMART framework (Specific, Measurable, Achievable, Relevant, Time-bound) ensures that your goals are actionable and trackable over time. For instance, if customer retention is your main focus, you could define it as follows:
🎯 Short-term goals: Analyze customer purchasing behavior and feedback over the next three months to identify the reasons behind declining retention rates.
📈 Medium-term goals: Increase customer retention by 10% within six months by implementing targeted strategies based on data insights.
🏆 Long-term goals: Build sustainable customer relationships and position your brand as a trusted leader in home appliances.
Once your goals are clearly defined, it’s crucial to consider the channels from which you’ll be collecting data. Manual data collection can be tedious and time-consuming, so it's essential to leverage a solution that enables you to analyze both customer interactions and unstructured customer data from various communication channels.
Step 2: Collect data
Once you’ve defined your goals, the next step is to gather data from various sources to get a holistic view of your customers. Collecting diverse data points will enable deeper insights into customer engagement and behavior. Key data sources include:
🛒 Purchase data: Track past purchases, order frequency and average order value to identify buying patterns.
🌐 Website and app analytics: Monitor customer navigation, time spent on pages and cart abandonment rates to evaluate the digital customer journey.
📞 Customer support interactions: Here, you can analyze call logs, chat transcripts and support tickets to confidently uncover recurring issues or service challenges, reassuring your understanding of customer needs.
💬 Social media interactions: Extract feedback from customer comments, reviews and mentions to gauge sentiment and understand broader customer preferences.
📝 Surveys and feedback forms: Collect direct feedback on product quality, customer satisfaction and service efficiency to align with customer expectations.
✅ Speaking of surveys...
Speaking of surveys, advanced online survey software has transformed how businesses gather customer feedback, creating a comprehensive 360° view. This software seamlessly integrates survey responses from social and digital channels, review sites and service interactions, simplifying setup and eliminating siloed data and manual workflows.
With these tools, you can extract patterns and themes from open-ended questions and employ statistical techniques like correlation and regression analyses to uncover relationships within the data. Additionally, you can ensure stakeholders receive relevant insights through role-based reporting, ensuring no critical feedback slips through the cracks.
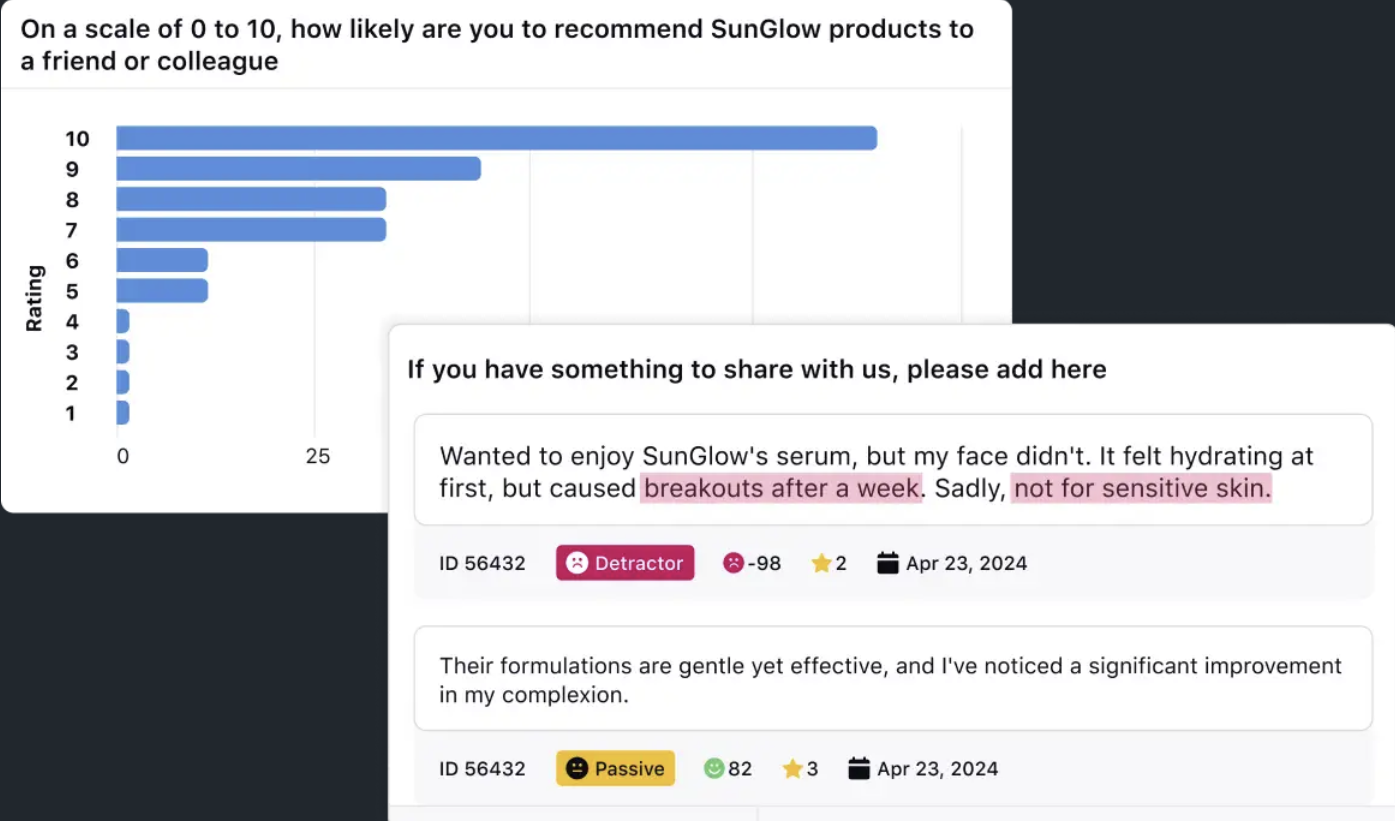
Step 3: Clean the data for accuracy
Raw data is often messy and inconsistent, so cleaning and organizing it is a crucial step before moving into deeper analysis. A well-maintained dataset ensures accuracy and actionable insights. Here’s how you can clean the data:
🗑️ Remove duplicates: Eliminate redundant records, such as duplicate accounts or repeat entries, to avoid skewed results.
✏️ Fix incomplete or inaccurate entries: Correct missing customer details, purchase history gaps or incorrect information that could impact data quality.
📊 Standardize data formats: Ensure consistency across data points — such as dates, names and product categories—to make analysis easier and more reliable.
A cleaned and organized dataset reduces error rates and helps build trust in your analytics, which is vital for making informed decisions.
Step 4: Categorize data for deeper insights
To make sense of your cleaned data, it’s important to categorize and segment it into meaningful groups. Segmentation helps you identify specific patterns and tailor strategies accordingly. Key segments could include:
👥 Customer segments: Group customers by factors such as demographics, purchase frequency or lifetime value to understand how different customer profiles behave. Read more about customer segmentation.
📦 Product segments: Organize data by product types to analyze which offerings drive repeat purchases or are underperforming.
🛠️ Feedback categories: Sort customer feedback into categories like product issues, service quality or delivery concerns to identify common pain points.
Segmenting your data ensures that you can target specific areas for improvement and create more personalized marketing, support and product strategies.
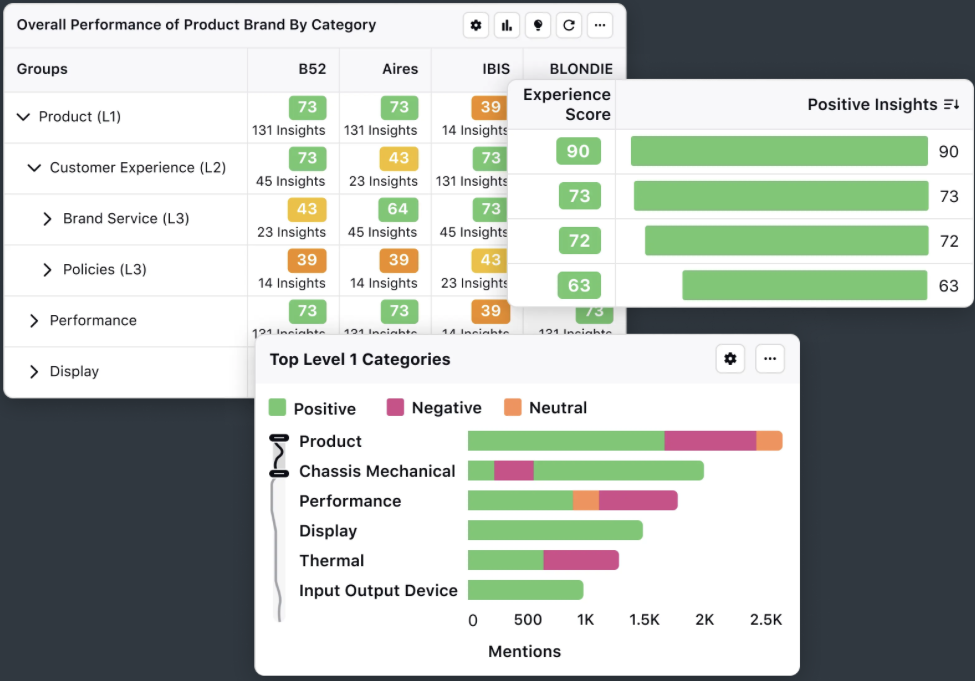
Step 5: Identify key patterns
With your data now cleaned, organized, and segmented, the next step is to dive into the analysis to uncover key trends and actionable insights. The goal here is to identify patterns that align with your goals, such as customer retention:
🛒 Purchase behavior patterns: For instance, customers who frequently buy high-ticket items may not return quickly due to the long lifecycle of the product, whereas customers of lower-priced items may be more likely to make repeat purchases.
🌐 Website interaction patterns: High cart abandonment rates may point to a confusing checkout process or slow load times driving customers away.
🔄 Retention patterns: Customers who receive proactive service after their purchase tend to show higher retention rates and increased brand loyalty.
🤝 Customer support trends: Recurrent complaints about specific issues, like delivery delays, may highlight operational inefficiencies that need to be addressed to improve the overall experience.
However, modern conversational analytics software eliminates the guesswork entirely.
When experiencing high call volumes or a surge in customer support tickets, advanced conversational analytics software can help you dig deeper into each contact driver. These tools identify the primary reasons behind customer inquiries and reveal the underlying root causes in detail. Furthermore, they provide actionable recommendations, enabling you to address these issues effectively and efficiently.
The true advantage lies in analyzing every interaction across all customer service channels — from voice calls to chat messages to unstructured public data across 30+ social and digital platforms. This 360-degree view of customer interactions allows you to gather rich, actionable insights and respond proactively to customer needs.
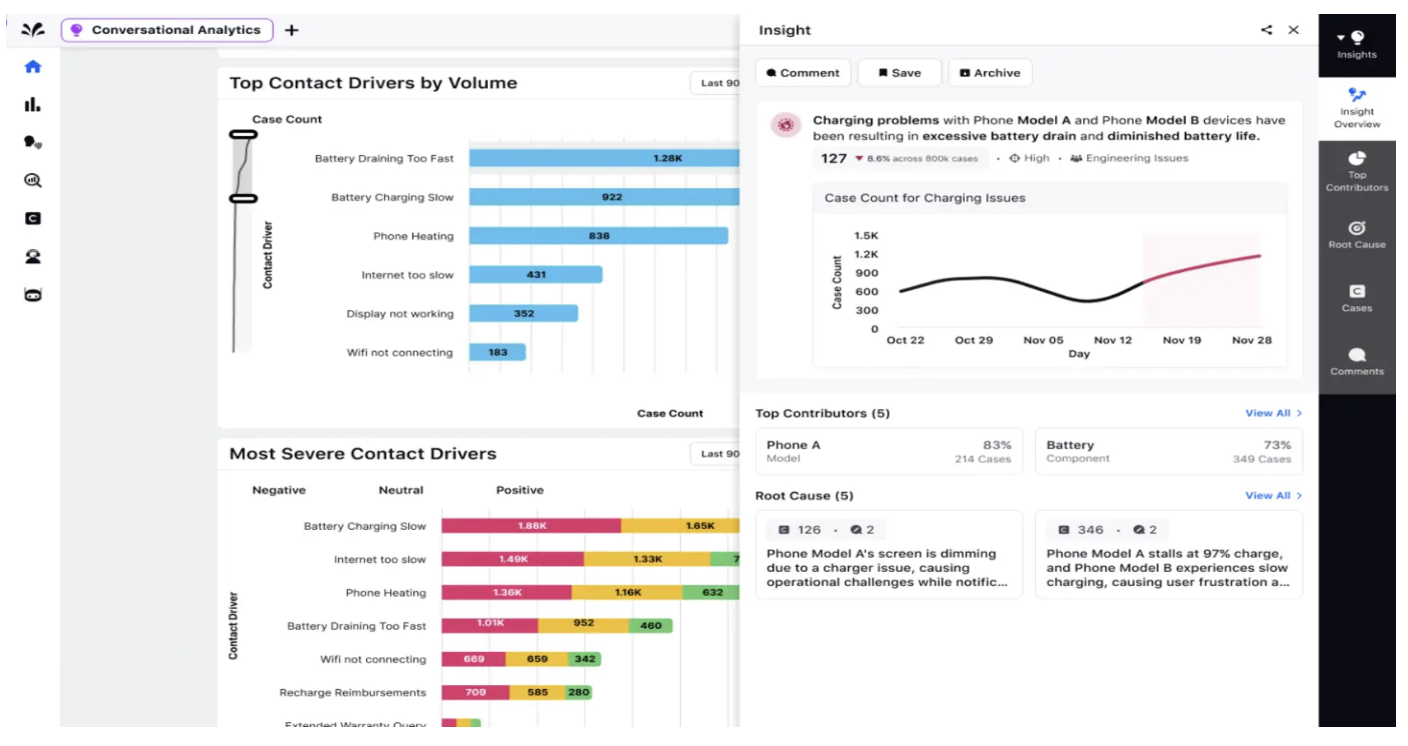
Step 6: Implement insights and measure success
The final step is putting your analysis to work. This involves developing strategies based on your gathered consumer insights and regularly measuring their success to ensure you’re achieving your goals. Here’s how:
📈 Develop targeted strategies: Based on the patterns you’ve uncovered, implement initiatives such as personalized marketing campaigns, improved customer service protocols, or product enhancements to address the issues or opportunities identified.
🛠️ Use automation tools: Leverage AI and automation tools to monitor real-time data and adjust strategies as needed continually. For example, automated customer feedback loops can help you address issues as soon as they arise.
🎯 Measure and iterate: Use key performance indicators (KPIs) aligned with your original goals to track the effectiveness of your strategies over time. Adjust your approach based on the data to ensure continuous improvement.
By regularly revisiting and refining your strategies, you ensure that your customer analytics efforts drive tangible, long-term improvements in retention and overall customer satisfaction.
Categories of customer analytics
Customer analytics helps understand behavior, diagnose issues, predict outcomes and recommend actions. Here are some common categories:
1. Descriptive analytics
Descriptive analytics collects and reviews past and present data to analyze customer actions. It helps you identify patterns or trends in specific customer segments.
For example, a fashion brand might use descriptive analytics to track seasonal requirement trends for a product such as winter coats, helping it optimize marketing strategies and inventory management.
2. Diagnostic analytics
Diagnostics analytics dives deeper into the data to uncover the reasons behind customer behavior. It helps you understand the “why” by examining the internal and external factors.
Imagine this: a brand sees a sudden sales drop in a region. Diagnostic analytics can identify the cause, such as supply chain disruptions affecting product availability in that area.
3. Predictive analytics
Predictive analytics forecasts future customer behavior using AI and statistical methods to analyze trends. It helps you anticipate how customers react and plan strategic moves in response.
A travel agency might predict summer vacation bookings by analyzing past travel patterns and customer preferences. This would allow the agency to market popular holiday destinations with tailored messages.
4. Prescriptive analytics
Prescriptive analytics goes further by recommending specific actions based on the data. It helps identify the best action to achieve desired outcomes, such as preventing customer churn and improving customer engagement.
Suppose an airline predicts weather delays; prescriptive analytics can recommend rebooking passengers and offering travel vouchers to maintain satisfaction.
Types of customer analytics
Different types of customer analytics allow you to gather valuable insights and provide tailored solutions to your clients. Let’s examine further:
1. Customer journey analytics
Customer journey analytics helps you understand how customers interact with your brand at various stages — from initial research to post-purchase. It tracks the customer’s path across multiple touchpoints.
For example, a furniture retailer might use journey analytics to track customer cart abandonment and identify areas for improvement.
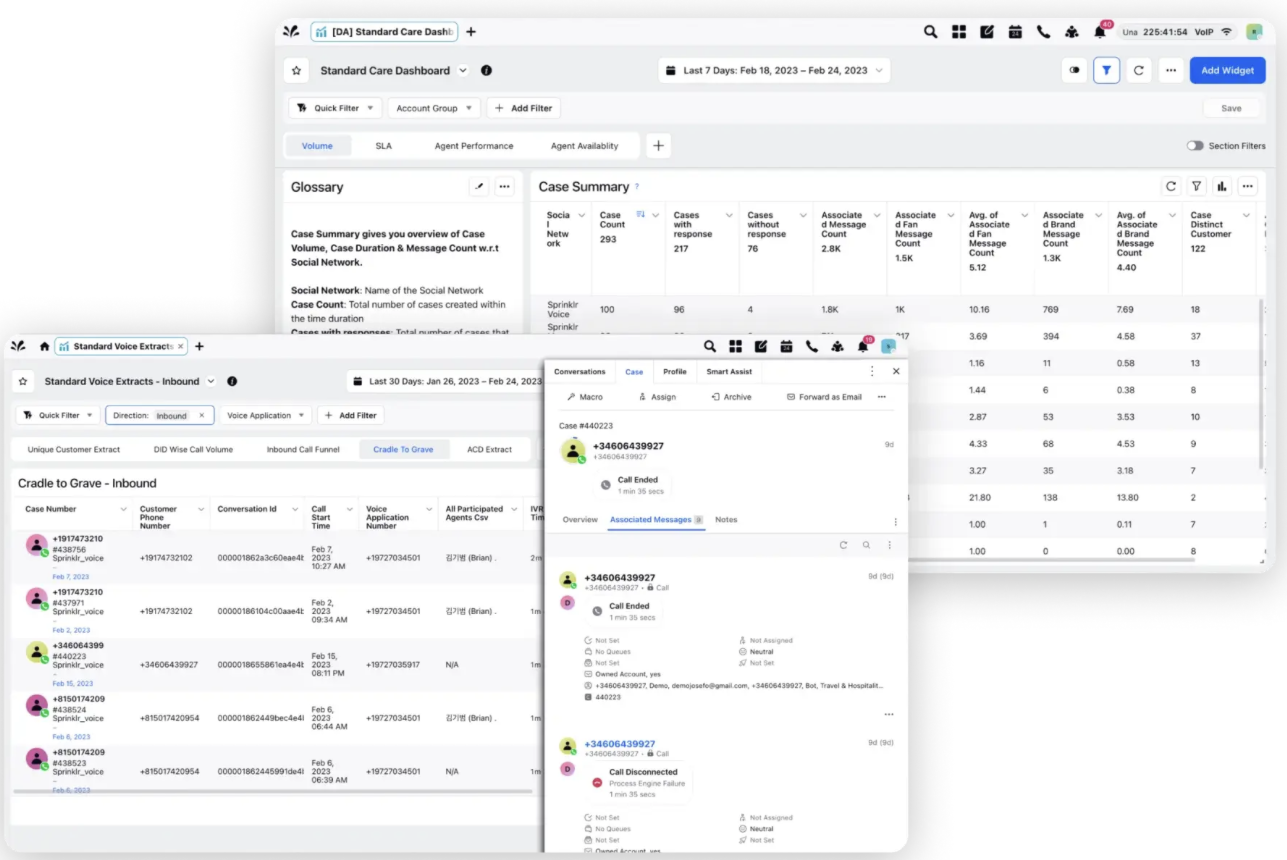
2. Customer experience analytics
Customer experience analytics gives businesses a broader understanding of how customers feel and interact with their brands. It identifies pain points and specifies improvement solutions.
An airline could use customer experience analytics to review post-flight feedback, identify issues like delayed service or uncomfortable seating, improving passenger satisfaction.
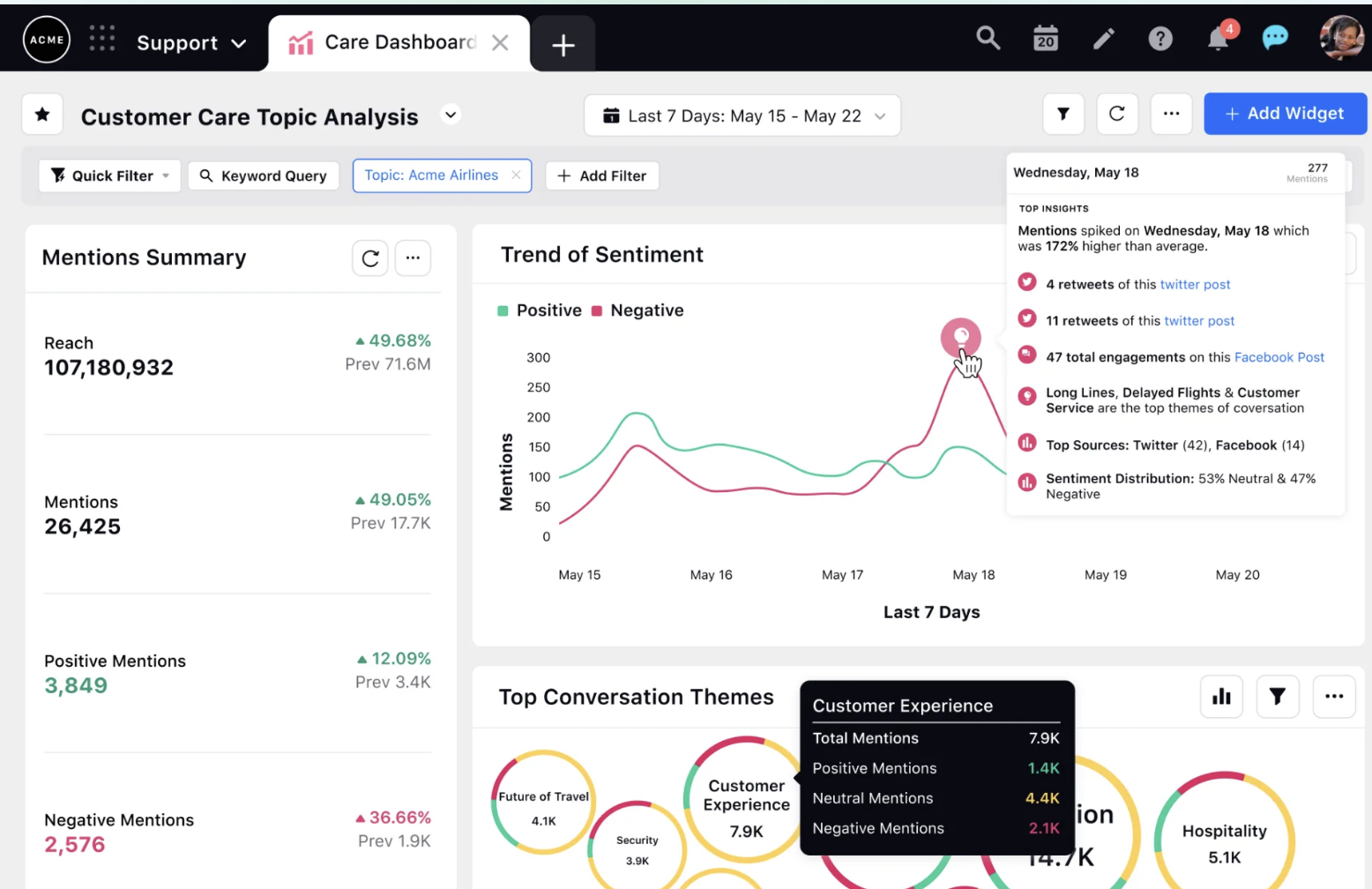
3. Customer engagement analytics
It tracks every product, service or brand interaction. It helps you understand customer behavior and predict future engagement.
A food chain might use engagement analytics to track mobile app usage and improve customer retention by offering personalized promotions and rewards through the app.
4. Customer loyalty or retention analytics
Loyalty or retention analytics focuses on long-term engagement. It helps you understand repeat purchase behavior and churn rates. So, you can tailor strategies to keep up with customer expectations and improve retention.
An FMCG company might leverage loyalty analytics to segment customers into frequent, occasional and rare buyers, tailoring its marketing to boost engagement in each group.
5. Voice of customer (VOC) analytics
VoC captures customer feedback, preferences and perceptions about a brand’s products or services. It provides a clear view of customer needs and helps you take action.
For instance, an insurance company might use the voice of customers to gather feedback on its claims process and policy renewals. It could then leverage those insights to streamline its claims procedures and enhance customer service.
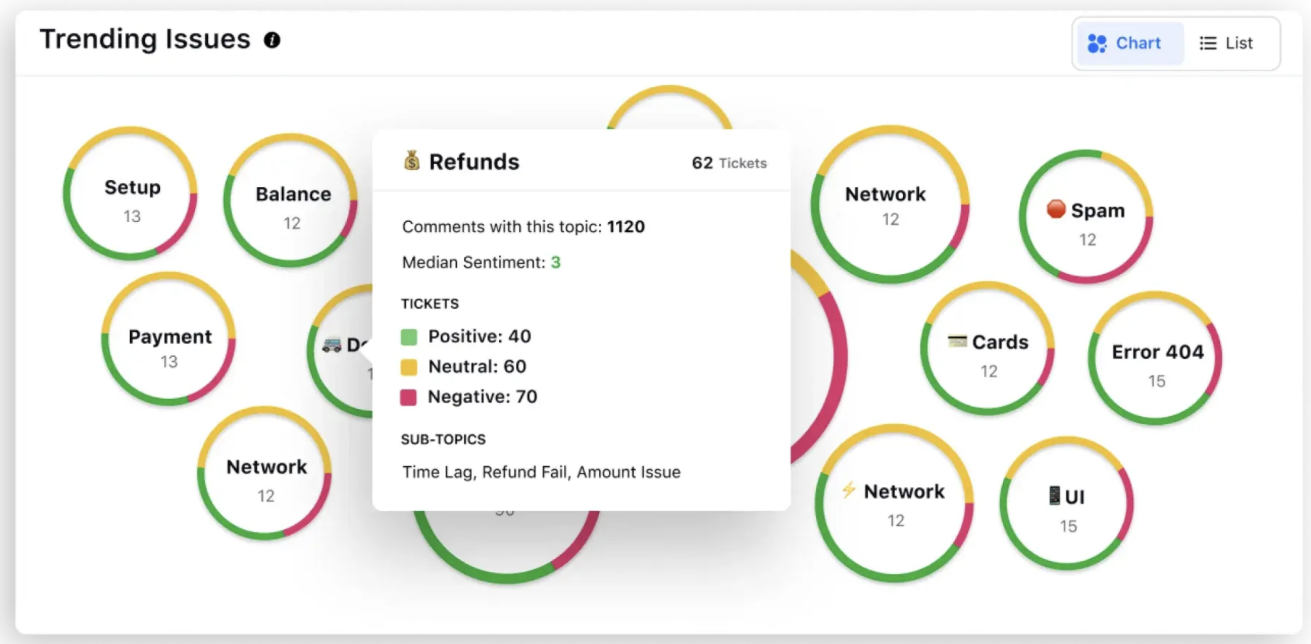
6. Customer sentiment analytics
Sentiment analytics focuses on analyzing customer feedback from sources like reviews, social media, surveys and emails. This helps you understand customer emotions (positive, negative or neutral) about your products or services, including attributes.
For example, a telecom company can gauge its customer sentiment against a range of attributes such as network coverage, app performance, internet speed, billing and more.
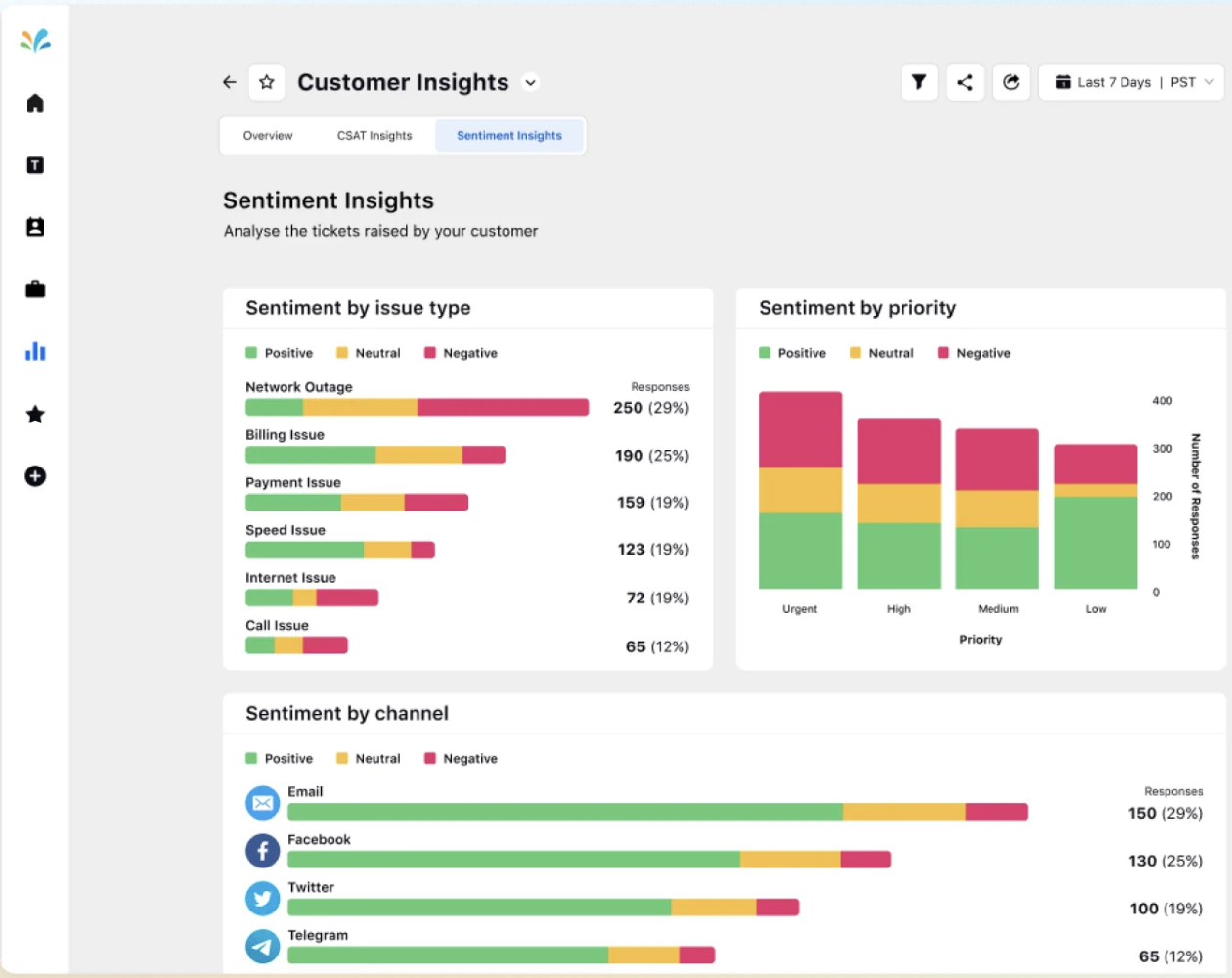
Why do businesses need customer analytics?
Here are key benefits demonstrating how customer analytics drives business success.
📣 Personalized marketing
Reports suggest that 63% of customers expect personalized engagement, so targeting specific segments with tailored messaging is essential. Customer analytics enables you to segment audiences and create personalized advertising campaigns.
🔄 Improved customer retention
A study shows that increasing retention rates by just 5% can lead to a 25% to 95% increase in profits. Customer analytics helps you spot patterns that indicate customer loyalty or churn risks.
🛍️ Enhanced customer experience
Customer analytics can uncover pain points you might not be aware of in the customer journey. Knowing the issues and proactively addressing them improves the overall customer experience.
📈 Increased sales and revenue
According to Forbes, 48% of customers are willing to pay more for quality customer service. Customer analytics gives you valuable insights into customers' purchase patterns, preferences and expectations. By leveraging these insights, you can enhance customer service and boost sales and revenue growth.
📉 Reduced customer acquisition costs
Customer analytics helps you add value at every customer journey stage, leading to more closed sales and longer customer retention. This efficiency significantly reduces customer acquisition costs, promoting sustainable growth. In fact, companies that use customer analytics intensively are 23 times more likely to acquire new customers faster than their competitors.
Key metrics associated with customer analytics
To make the most of customer analytics, it’s important to track the right metrics that provide insights into customer behavior, satisfaction and engagement. Here are some of the most critical metrics to consider:
1. Customer lifetime value (CLV):
This metric estimates the total expected revenue from a single customer over their relationship with the brand.
APV - (Average purchase value)
2. Customer retention rate (CRR):
This metric shows the percentage of customers associated with your product or services over a specific period.
3. Churn rate:
It measures the percentage of customers who stop using a product or service during a given period.
4. Net promoter score (NPS)
NPS gauges customer loyalty and their likelihood of recommending your product or service to others. Calculating NPS requires a business to ask their customers a simple question: "On a scale of 0-10, how likely are you to recommend us to a friend or colleague?" The respondents are categorized into three groups:
Promoters: those who respond with 9 or 10, indicating a high likelihood of recommendation.
Passives who respond with 7 or 8 are considered neutral and not factored into the NPS calculation.
Detractors: who respond with 0 to 6, indicating a low likelihood to recommend.
📚 Read More: How to improve the Net Promoter Score of your brand
5. Customer satisfaction score (CSAT):
CSAT measures the level of satisfaction customers feel after interacting with your brand. It’s typically collected via surveys following specific touchpoints, such as a purchase or a customer service interaction.
6. Average order value (AOV):
AOV calculates the average amount customers spend on each transaction. By tracking AOV, you can gauge purchasing behavior and determine which strategies—such as cross-selling or promotional offers—are most effective in increasing order sizes.
7. Time to resolution:
It tracks the average time it takes for your support team to resolve customer issues. A lower TTR can lead to higher satisfaction, as customers appreciate quick and effective solutions to their problems.
Industry-specific use cases of customer analytics
Let’s look at how different sectors leverage customer analytics:
🛒 E-commerce: Retailers use customer analytics to identify popular products, adjust pricing and provide personalized recommendations. By analyzing browsing history and reviews, e-commerce platforms can enhance marketing, reduce cart abandonment and boost sales and customer satisfaction.
For instance, Walmart's implementation of data analytics revolutionized its operations, enhancing inventory management, boosting customer satisfaction and driving profitability.
⚕️ Healthcare: Healthcare providers utilize data analytics to examine patient demographics, treatment effectiveness and interactions with health services. This allows them to customize care plans and proactively address emerging health trends, leading to better patient care and improved outcomes.
Corewell Health researchers highlighted how predictive analytics enabled the organization to prevent 200 patient readmissions and achieve $5 million in cost savings.
🏦 Financial services: Banks and financial institutions leverage customer analytics to assess credit risk, detect fraud and offer personalized financial products. By analyzing transaction history, spending and saving habits, banks can offer more accurate loan terms and detect fraudulent activities more effectively.
For example, a European bank used machine learning to identify active customers likely to reduce their business to address a shrinking customer base. This insight from predictive analytics led to a targeted campaign that cut churn by 15%.
The role of AI in customer analytics
Artificial intelligence plays a crucial role in enhancing the accuracy and efficiency of customer analytics, allowing you to act quickly and proactively. When deployed in customer analytics, AI can -
📊 Deliver automated insights: AI-generated insights help you quickly identify patterns in customer behavior. For instance, AI can predict customer churn or uncover upsell opportunities, assisting brands in taking timely action.
🗣️ Enable customer sentiment monitoring: AI analyzes customer interactions across channels to analyze sentiment in real time. This allows you to respond swiftly to customer complaints, improving customer satisfaction and bolstering brand reputation.
📊 Offer advanced analytics: AI-driven predictive and prescriptive analytics offer deeper insights, such as predicting loan defaults or identifying cross-selling opportunities, enabling you to make smarter strategic decisions and achieve desired outcomes faster and more effectively.
📚 Related Read: How to Better Integrate AI, CX and Data to Enhance Business Growth
How to get started with customer analytics:
Today, simply collecting data isn't enough. The real challenge lies in transforming that data into actionable insights to solve critical pain points such as declining customer retention, operational inefficiencies, and lack of personalized service. Without a clear understanding of the patterns and trends hidden within customer data, you risk losing valuable customers to competitors more attuned to their needs.
Customer retention, in particular, is a complex puzzle that involves understanding customer behaviors across multiple touchpoints — from purchase history to website interactions and customer support experiences. Many businesses face difficulty identifying the root causes behind churn, missed opportunities and customer dissatisfaction, leading to fragmented service experiences.
This is where Sprinklr's AI-powered reporting and analytics comes in. With Sprinklr, you can gain a 360° view of customer interactions across social, digital and traditional channels. Here's what else you can unlock:
AI-powered insights: Sprinklr AI can automatically identify key patterns and trends in your customer data, whether from purchase behavior, website interaction or support tickets.
Real-time analytics: By analyzing customer interactions in real-time, Sprinklr allows you to predict customer behavior and intervene proactively before issues escalate.
End-to-end data integration: Say goodbye to data silos. Sprinklr integrates data from all touchpoints — a social media review, a customer support call or a product feedback survey — to get a unified view of every customer journey.
Actionable recommendations: Sprinklr provides actionable recommendations based on AI-driven insights. Whether optimizing your checkout process or identifying the root cause of customer complaints, Sprinklr ensures you are always a step ahead.
For more information, schedule a demo with our experts today!
FAQ
Thank you for contacting us.
A Sprinklr representative will be in touch with you shortly.
Contact us today, and we'll create a customized proposal that addresses your unique business needs.
Request a Demo
Welcome Back,
No need to fill out any forms — you're all set.